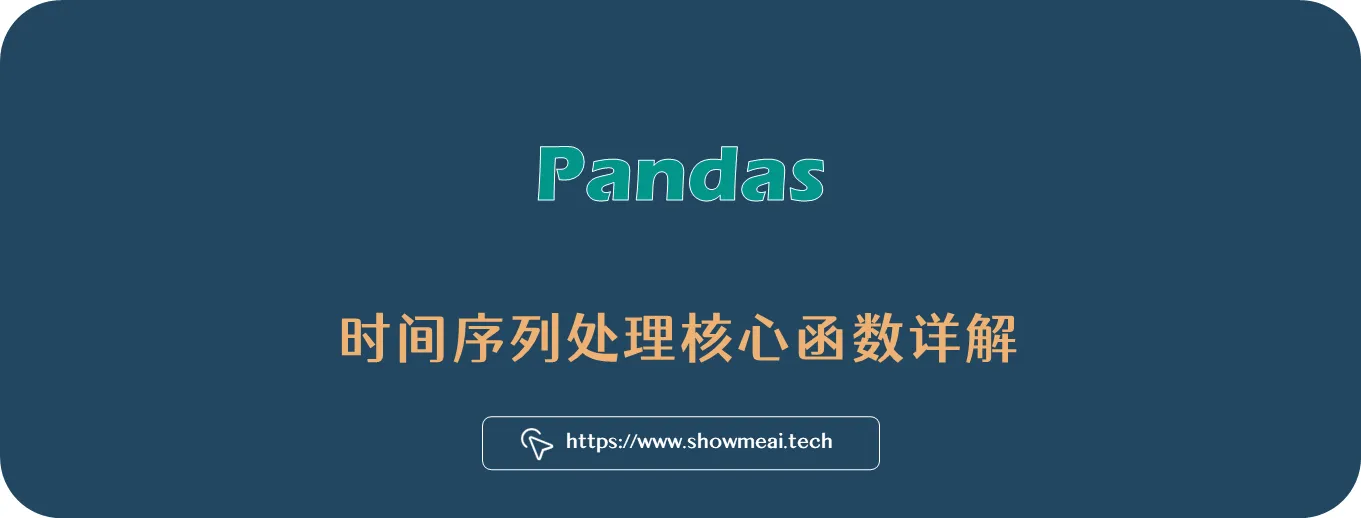
💡 作者: 韩信子@ ShowMeAI
📘 数据分析实战系列: https://www.showmeai.tech/tutorials/40
📘 本文地址: https://www.showmeai.tech/article-detail/389
📢 声明:版权所有,转载请联系平台与作者并注明出处
📢 收藏 ShowMeAI查看更多精彩内容
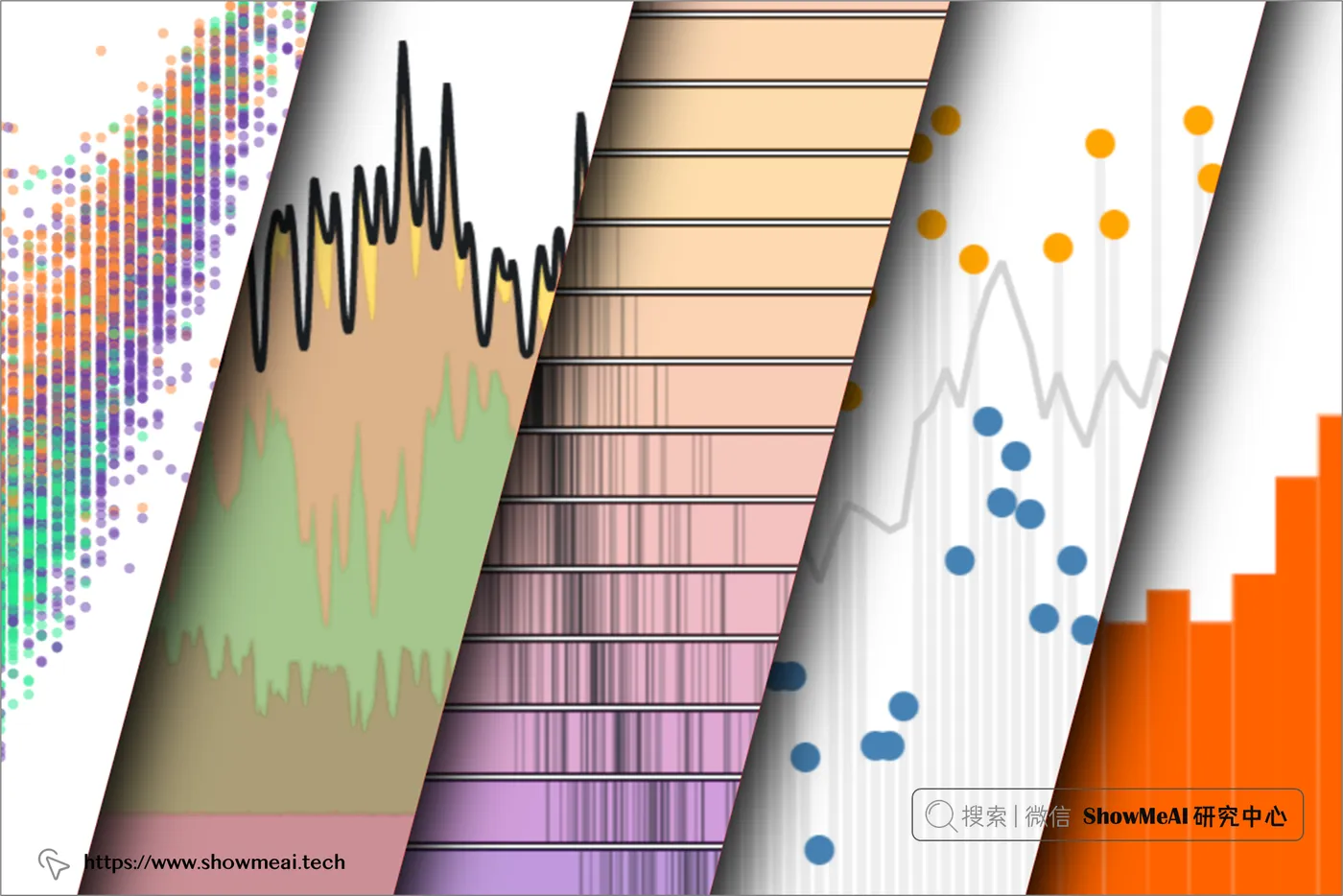
Pandas 是大家都非常熟悉的数据分析与处理工具库,对于结构化的业务数据,它能很方便地进行各种数据分析和数据操作。但我们的数据中,经常会存在对应时间的字段,很多业务数据也是时间序组织,很多时候我们不可避免地需要和时间序列数据打交道。其实 Pandas 中有非常好的时间序列处理方法,但是因为使用并不特别多,很多基础教程也会略过这一部分。
在本篇内容中,ShowMeAI对 Pandas 中处理时间的核心函数方法进行讲解。相信大家学习过后,会在处理时间序列型数据时,更得心应手。
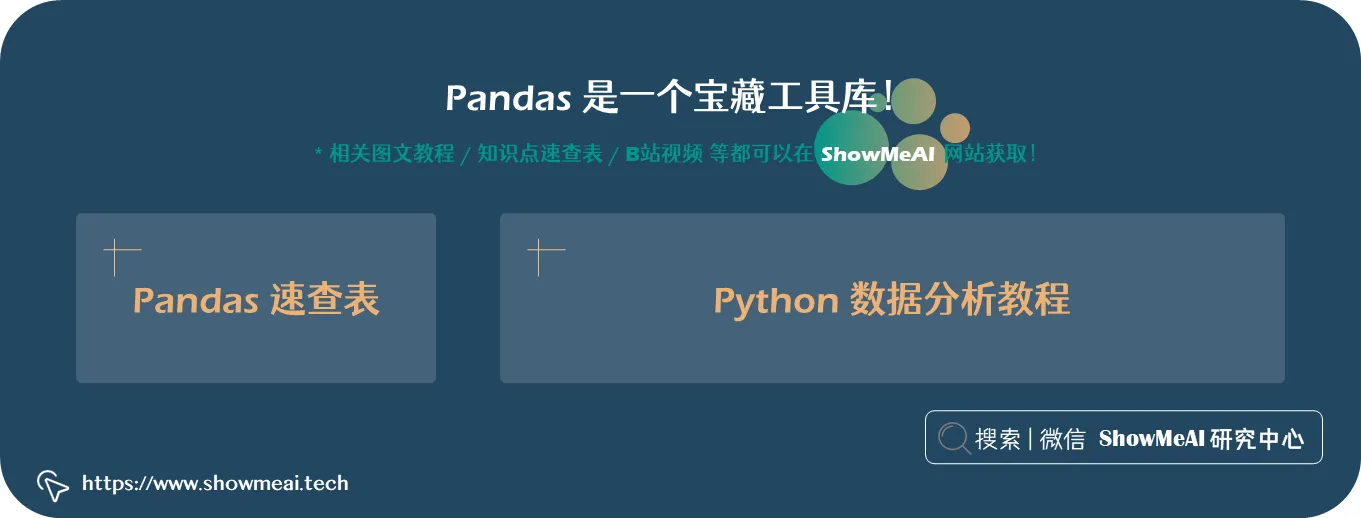
数据分析与处理的完整知识技能,大家可以参考 ShowMeAI制作的工具库速查表和教程进行学习和快速使用。
💡 时间序列
时间序列是指将同一统计指标的数值按其发生的时间先后顺序排列而成的数列。简单说来,时间序列是随着时间的推移记录某些取值,比如说商店一年的销售额(按照月份从1月到12月)。
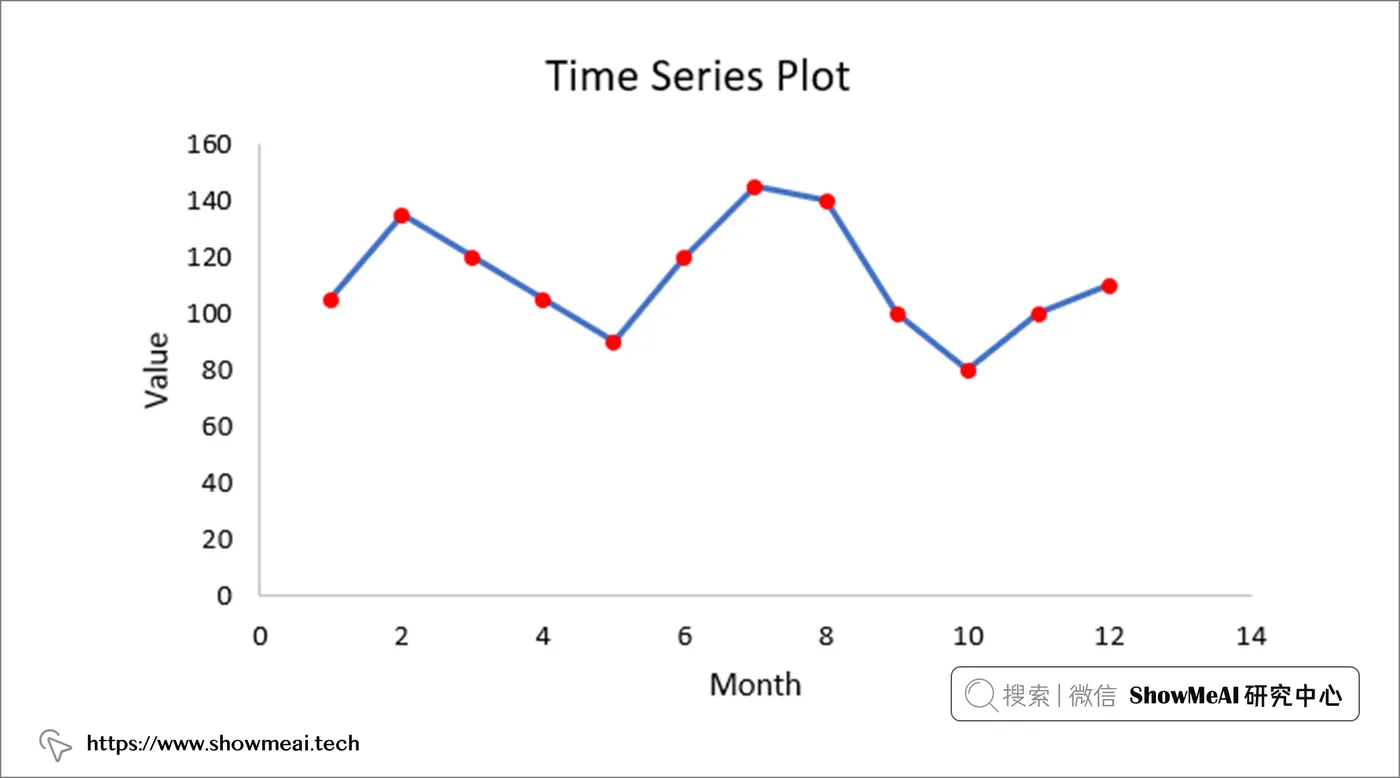
💡 Pandas 时间序列处理
我们要了解的第一件事是如何在 Pandas 中创建一组日期。我们可以使用date_range()
创建任意数量的日期,函数需要你提供起始时间、时间长度和时间间隔。
# 构建时长为7的时间序列
pd.date_range("2022-01-01", periods=7, freq='D')
# 输出
# DatetimeIndex(['2022-01-01', '2022-01-02', '2022-01-03', '2022-01-04','2022-01-05', '2022-01-06', '2022-01-07'], dtype='datetime64[ns]', freq='D')
注意到上面的频率可用freq
来设置:最常见的是'W'
每周,'D'
是每天,'M'
是月末,'MS'
是月开始。
下面我们创建一个包含日期和销售额的时间序列数据,并将日期设置为索引。
# 设置随机种子,可以复现
np.random.seed(12)
# 构建数据集
df = pd.DataFrame({
'date': pd.date_range("2022-01-01", periods=180, freq='D'),
'sales': np.random.randint(1000, 10000, size=180)})
# 设置索引
df = df.set_index('date')
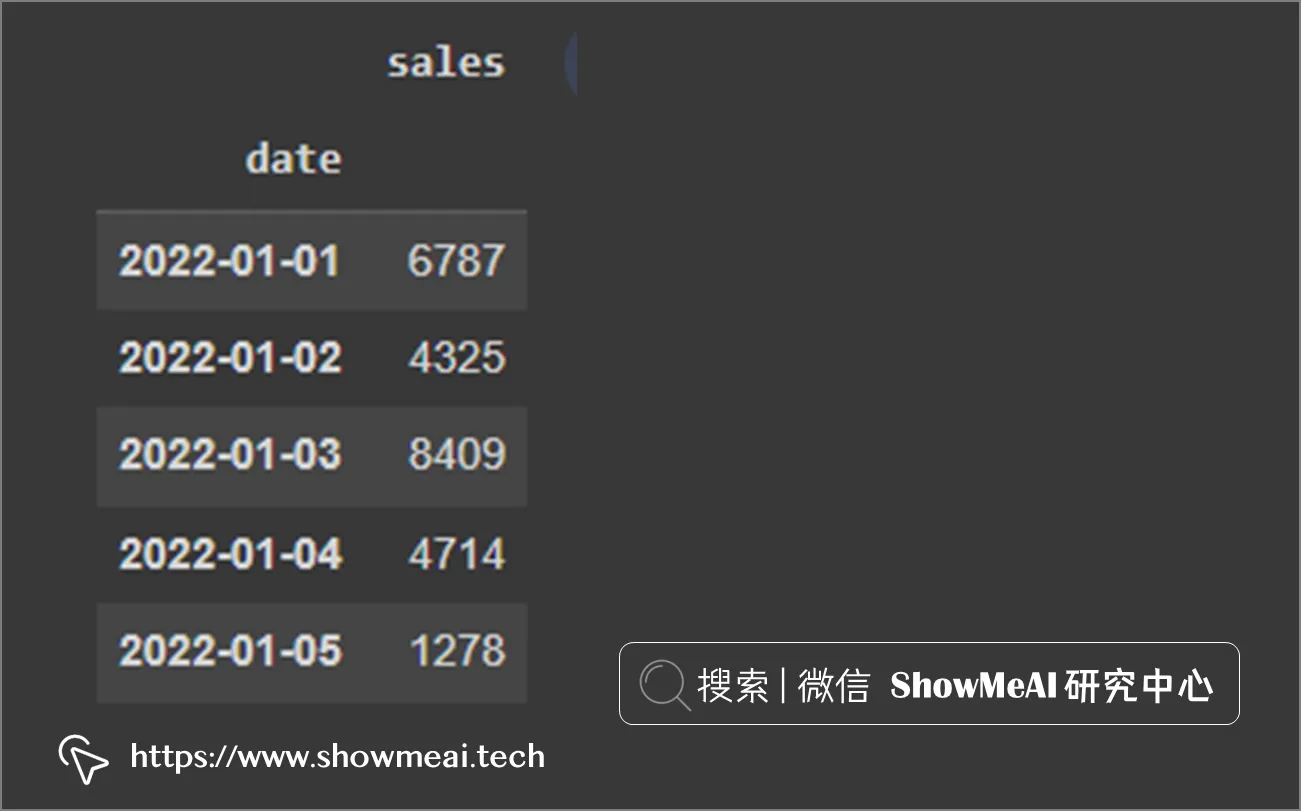
注意,我们要方便地对时间序列进行处理,一个很重要的先序工作是将日期作为索引,我们前面已经完成这个工作了。
💦 重采样
Pandas 中很重要的一个核心功能是resample
,重新采样,是对原样本重新处理的一个方法,是一个对常规时间序列数据重新采样和频率转换的便捷的方法。
方法的格式是:
DataFrame.resample(rule, how=None, axis=0, fill_method=None, closed=None, label=None, convention='start',kind=None, loffset=None, limit=None, base=0)
核心的参数rule
是字符串,表示采样的频度。如下代码,在resample
后接的mean
是表示按照月度求平均。
# Resample by month end date
df.resample(rule= 'M').mean()
按月取平均值后,将索引设置为每月结束日期,结果如下。
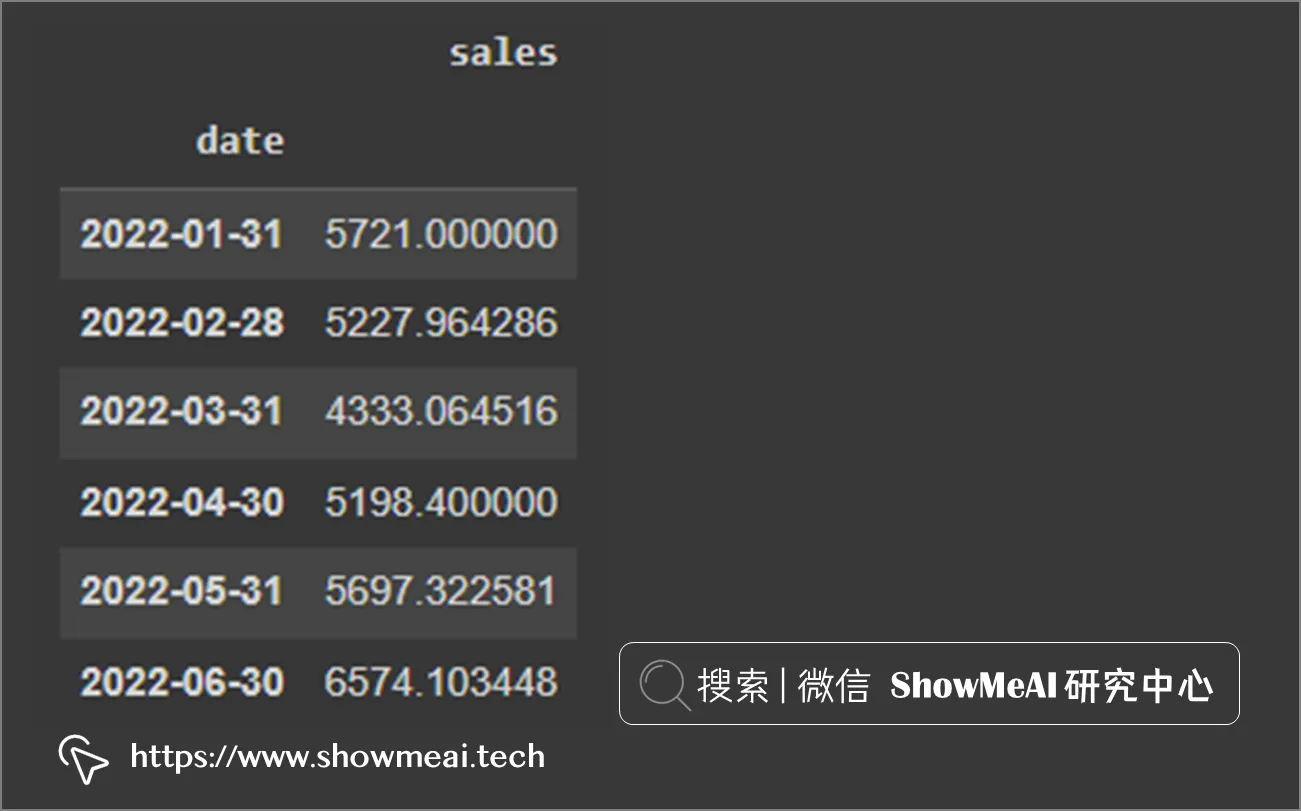
我们也可以按每周销售额绘制汇总数据。
# 采样绘图
df.resample('W').mean().plot(figsize=(15,5), title='Avg Weekly Sales');
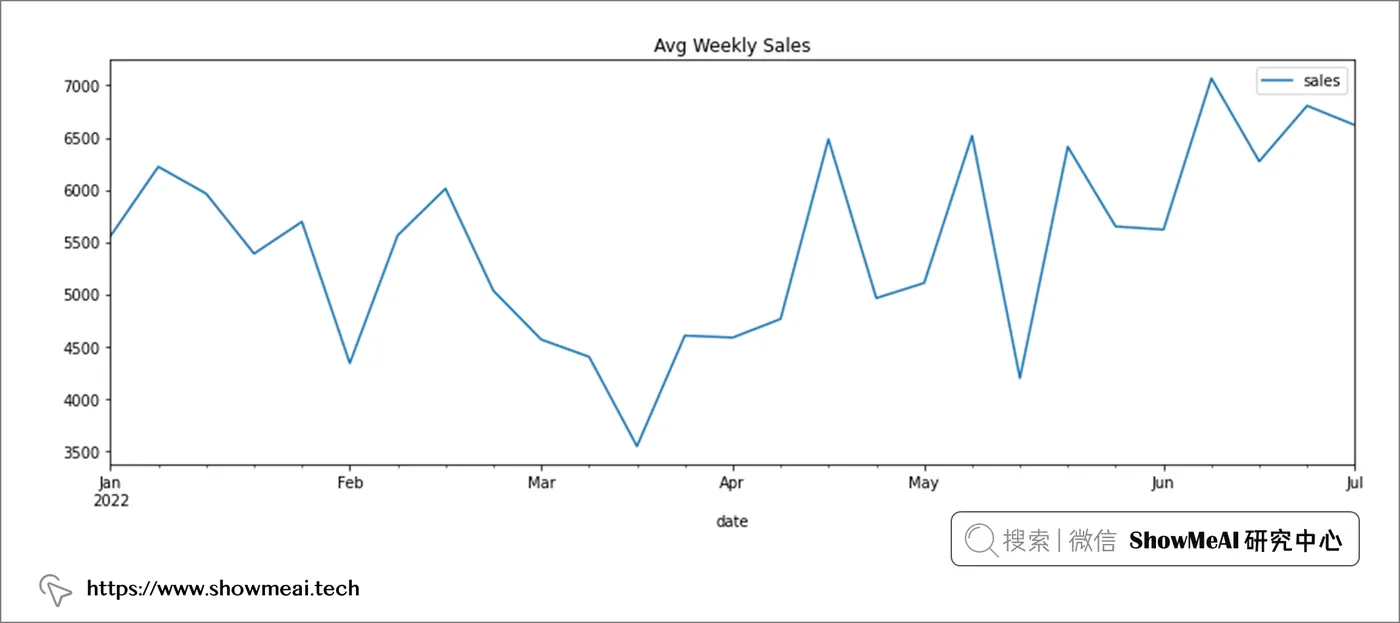
上图可以看出,销量在3月和4月之间的销售额有所下降,而在 6 月中旬达到顶峰。
💦 平移
Pandas 中的shift
功能,可以让字段向上或向下平移数据。这个平移数据的功能很容易帮助我们得到前一天或者后一天的数据,可以通过设置shift
的参数来完成上周或者下周数据的平移。
# 原始数据的一份拷贝
df_shift = df.copy()
# 平移一天
df_shift['next_day_sales'] = df_shift.sales.shift(-1)
# 平移一周
df_shift['next_week_sales'] = df_shift.sales.shift(-7)
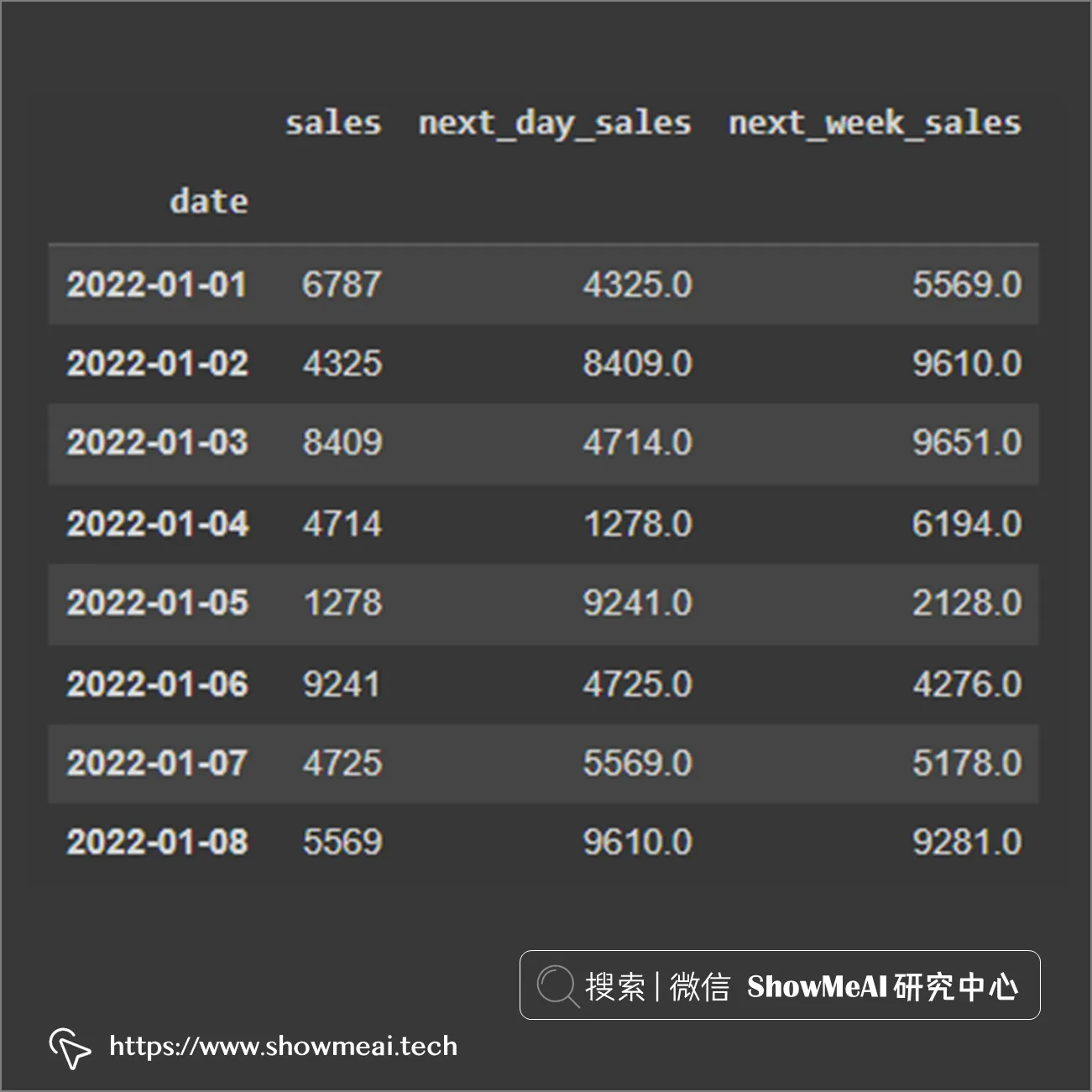
在时间序列问题中,我们经常要完成同比和环比数据,通过shift后的数据做差就很容易得到。
# 计算差值
df_shift['one_week_net'] = df_shift.sales - df_shift.sales.shift(-7)
💦 滑动平均
下一个核心功能是rolling
滑动平均,它是做交易的朋友非常常用到的一个功能,rolling
函数创建一个窗口来聚合数据。
# 长度为2天的窗口,求滑动平均
df.rolling(2).mean()
在下图中,我们可以看到第一个值是NaN
,因为再往前没有数据了。对第2个点,它对数据集的前2行计算平均: (6787 + 4325)/2 = 5556。
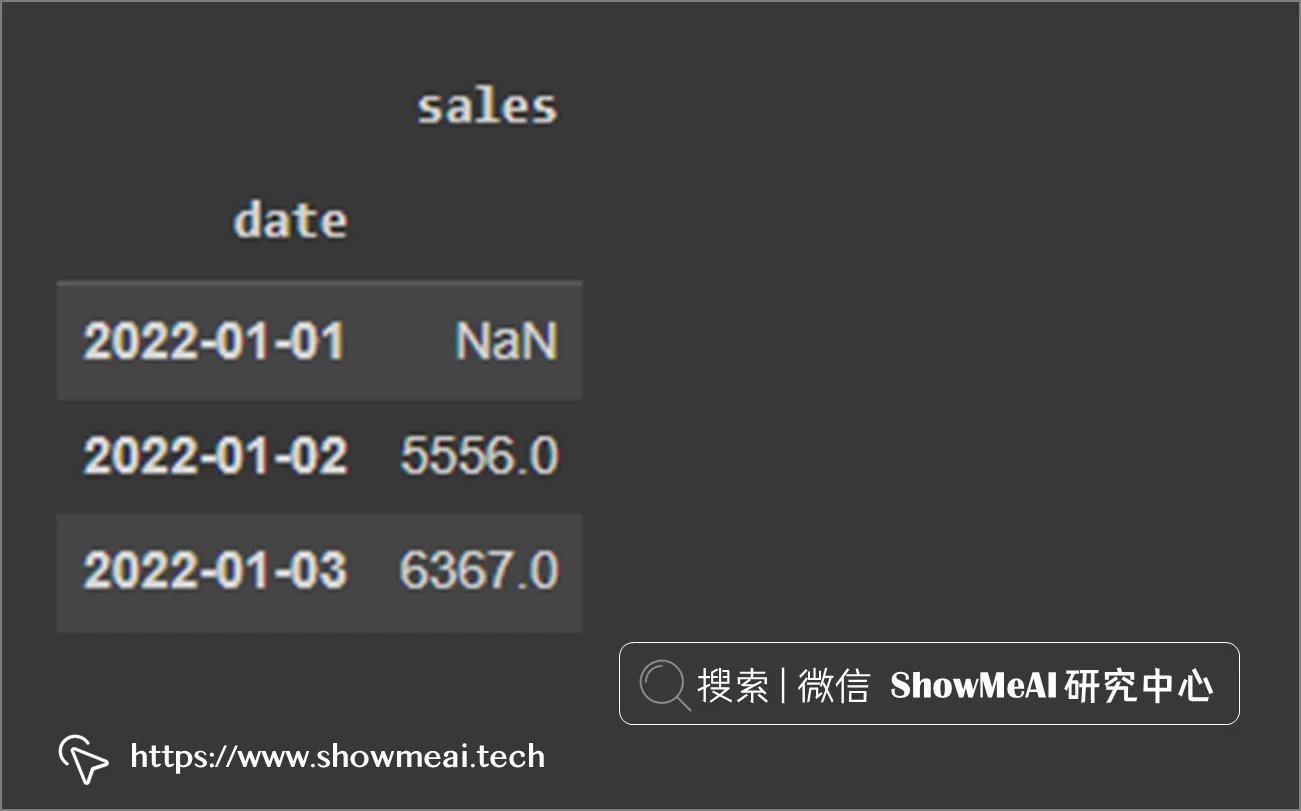
滚动平均值非常适合表征趋势,滑动窗口越大,得到的结果曲线越平滑,最常用的是7天平均。
# 滑动平均绘图
df.sales.plot(figsize=(25,8), legend=True, linestyle='--', color='darkgray')
df.rolling(window=7).sales.mean().plot(legend=True, label='7 day average', linewidth=2)
df.rolling(30).sales.mean().plot(legend=True, label='30 day average', linewidth=3)
df.rolling(100).sales.mean().plot(legend=True, label='100 day average', linewidth=4)
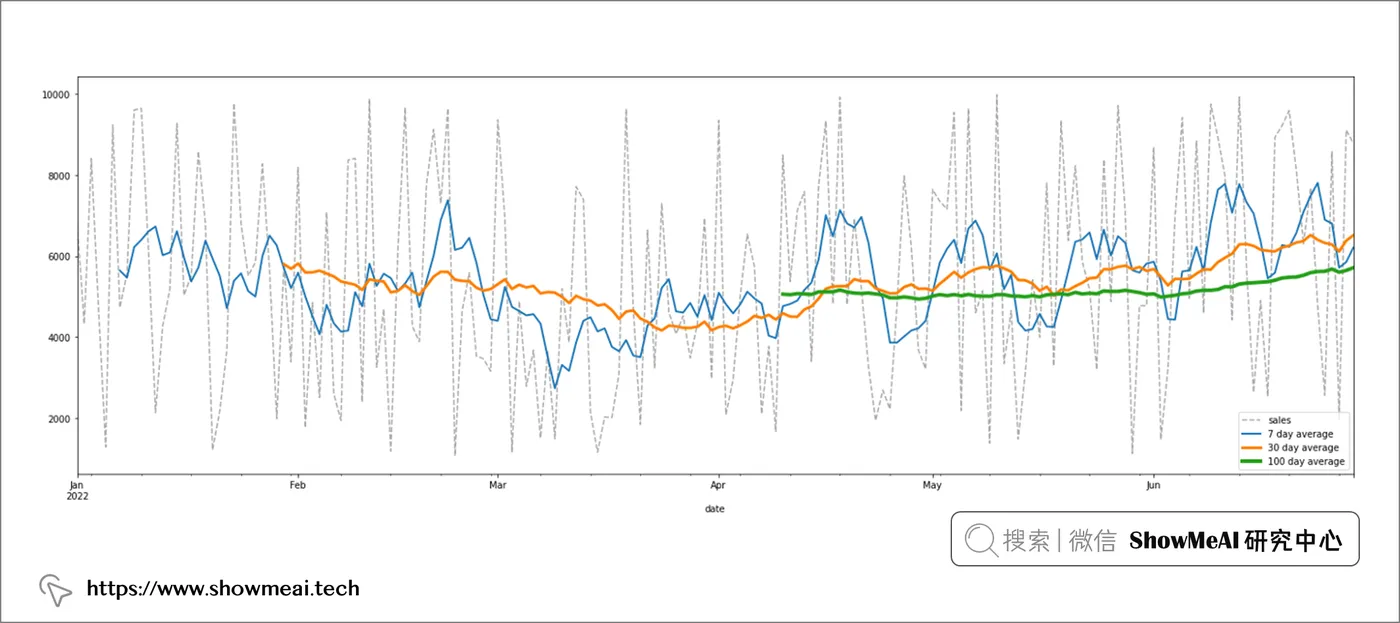
💡 总结
Pandas在时间序列处理和分析中也非常有效,ShowMeAI在本篇内容中介绍的3个核心函数,是最常用的时间序列分析功能:
resample
:将数据从每日频率转换为其他时间频率。shift
:字段上下平移数据以进行比较或计算。rolling
:创建滑动平均值,查看趋势。
参考资料
- 📘数据科学工具库速查表 | Pandas 速查表:https://www.showmeai.tech/article-detail/101
- 📘图解数据分析:从入门到精通系列教程:https://www.showmeai.tech/tutorials/33
推荐阅读
- 🌍 数据分析实战系列 :https://www.showmeai.tech/tutorials/40
- 🌍 机器学习数据分析实战系列:https://www.showmeai.tech/tutorials/41
- 🌍 深度学习数据分析实战系列:https://www.showmeai.tech/tutorials/42
- 🌍 TensorFlow数据分析实战系列:https://www.showmeai.tech/tutorials/43
- 🌍 PyTorch数据分析实战系列:https://www.showmeai.tech/tutorials/44
- 🌍 NLP实战数据分析实战系列:https://www.showmeai.tech/tutorials/45
- 🌍 CV实战数据分析实战系列:https://www.showmeai.tech/tutorials/46
- 🌍 AI 面试题库系列:https://www.showmeai.tech/tutorials/48