Factorization Machine---因子分解机
①target function的推导
logistics regression algorithm model中使用的是特征的线性组合,最终得到的分割平面属于线性模型,但是线性模型就只能处理线性问题,所以对于非线性的问题就有点难处理了,对于这些复杂问题一般是两种解决方法①对数据本身进行处理,比如进行特征转换,和函数高维扩展等等。②对算法模型本身进行扩展,比如对linear regression加上正则化惩罚项进行改进得到lasso regression或者是ridge regression。
Factorization Machine就是一种对logistics regression的一种改进,线性的部分权值组合是不变的,在后面增加了非线性的交叉项。
target function:表示的是系数矩阵V的第i维向量,
,k的大小称为是度,度越大代表分解出来的特征就越多。对于每一个特征都会对应有一个
维的向量。前两部分是传统的线性模型,后一个部分就是将脸刚刚互不相同的特征分量之间的相互关系考虑进来了。也就是不同特征之间的吸引程度。
如果使用男女恋爱来解释这个模型,得分score是男生对女生的一个喜欢程度,代表的就是底分,可以看成是男生对于女生的第一感觉。对于第二部分可以看成是女生的优秀程度,第三部分就相当于是男女之间的事交互关系了,也就是男女之间的感觉,如果两个男生对于同一个女生的感觉是一致的,那么他们的
就是一致的,从本质上说,因子分解机也是探索一种相似性,其与协同过滤算法是类似的,但是这两者的区别在于,因子分解机同时考虑了男生和男生间的相似性以及女生和女生间的相似性,但是协同过滤要么只考虑男生之间的相似性,要么只考虑女生之间的相似性。
优化求解target function
对于原始的target function计算复杂度是
,采用公式
的公式。于是化简一波:
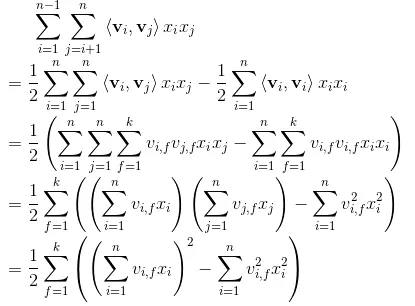
这样就成功的把复杂度降到了
FM可以解决的问题主要是四种:
1.回归问题:这时候的error function:
2.二分类问题:
3.排序问题。
4,推荐系统。
接下来就是模型的求解,自然就是求导了,我们做的是二分类问题,所以采用的就是第二种loss function求导,按照求导常规求取即可:
Factorization Machine的优点
①对于一些很稀疏的数据集也可以进行参数的预测,而SVM是不行的。
②FM有线性的复杂性,可以直接在原始数据进行预测,而不需要再做核函数或者特征转换,对于SVM,是要基于对支持向量的优化的。
③FMs是一种通用的预测器,可用于任何实值特征向量。相比之下。其他最先进的因数分解模型只在非常有限的输入数据上工作。通过定义输入数据的特征向量,FMs可以模拟最先进的模型,如偏置MF、SVD++、PITF或FPMC。
K的选择
如果数据是一个稀疏矩阵,那么可以选择一个比较小的k,因为稀疏矩阵其实就已经表明这个矩阵的信息是十分有限的了,再取比较大的k可能会导致过拟合。如果数据并不是一个稀疏矩阵,可以选择大一点的k来代表数据。
代码实现
主要部分的代码:
import numpy as np
import pandas as pd
import matplotlib.pyplot as plt
from loadDataSet import loadData
def Accuracy(preiction, classlabel):
score = 0
for i in range(len(preiction)):
if preiction[i] > 0.5:
preiction[i] = 1
else:
preiction[i] = -1
if preiction[i] == classlabel[i]:
score += 1
print('Accuracy: ', score/len(preiction))
def initialize(n, k):
v = np.mat(np.zeros((n, k)))
for i in range(n):
for j in range(k):
v[i, j] = np.random.normal(0, 0.2)
return v
def sigmoid(inx):
return 1.0/(1+np.exp(-inx))
def getCost(predict, classLabels):
m = len(predict)
error = 0.0
for i in range(m):
error -= np.log(sigmoid(predict[i]*classLabels[i]))
return error
def getPrediction(dataMatrix, w0, w, v):
m = np.shape(dataMatrix)[0]
result = []
for x in range(m):
inter_1 = dataMatrix[x] * v
inter_2 = np.multiply(dataMatrix[x], dataMatrix[x]) * np.multiply(v, v)
interaction = np.sum(np.multiply(inter_1, inter_1) - inter_2) / 2
p = w0 + dataMatrix[x] * w + interaction
pre = sigmoid(p[0, 0])
result.append(pre)
return result
def stocGradAscent(dataMatrix, classLabels, k, max_iter, alpha):
#initialize parameters
m, n = np.shape(dataMatrix)
w = np.zeros((n, 1))
w0 = 0
v = initialize(n, k)
#training
for it in range(max_iter):
for x in range(m):
inter_1 = dataMatrix[x] * v
inter_2 = np.multiply(dataMatrix[x], dataMatrix[x])*np.multiply(v, v)
interaction = np.sum(np.multiply(inter_1, inter_1) - inter_2)/2
p = w0 + dataMatrix[x]*w + interaction
loss = sigmoid(classLabels[x] * p[0, 0]) - 1
w0 = w0 - alpha*loss*classLabels[x]
for i in range(n):
if dataMatrix[x, i] != 0:
w[i, 0] = w[i, 0] - alpha*loss*classLabels[x]*dataMatrix[x, i]
for j in range(k):
v[i, j] = v[i, j] - alpha*loss*classLabels[x]*(dataMatrix[x, i]*inter_1[0, j]-v[i, j]*dataMatrix[x, i]*dataMatrix[x, i])
if it % 1000 == 0:
print('-----iter: ', it, ', cost: ', getCost(getPrediction(np.mat(dataMatrix), w0, w, v), classLabels))
Accuracy(getPrediction(np.mat(dataMatrix), w0, w, v), classLabels)
if __name__ == '__main__':
dataMatrix, target = loadData('../Data/testSetRBF2.txt')
stocGradAscent(dataMatrix, target, 5, 5000, 0.01)
AI 代码解读
准备数据部分:
import numpy as np
import pandas as pd
import matplotlib.pyplot as plt
def loadData(filename):
df = pd.read_csv(filename, sep=' ', names=['1', '2', 'target'])
data = []
target = []
for i in range(len(df)):
d = df.iloc[i]
ds = [d['1'], d['2']]
t = int(d['target'])
if t == 0:
t = -1
data.append(ds)
target.append(t)
return np.mat(data), np.mat(target).tolist()[0]
if __name__ == '__main__':
dataMatrix, target = loadData('../Data/testSetRBF2.txt')
print(dataMatrix)
print(target)
AI 代码解读
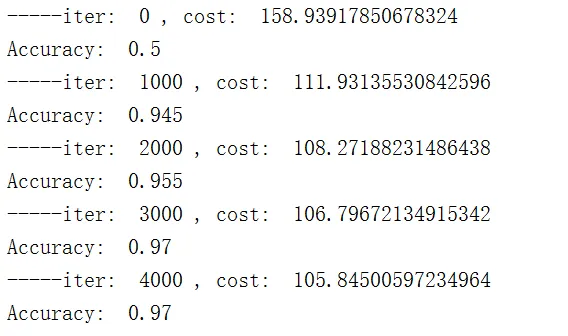
最后附上GitHub代码:
https://github.com/GreenArrow2017/MachineLearning/tree/master/MachineLearning/Factorization%20Machine