一、ViT-S/14
import torch
import torchvision.transforms as T
import matplotlib.pyplot as plt
import numpy as np
import matplotlib.image as mpimg
from PIL import Image
from sklearn.decomposition import PCA
import matplotlib
patch_h = 28
patch_w = 28
feat_dim = 384
transform = T.Compose([
T.GaussianBlur(9, sigma=(0.1, 2.0)),
T.Resize((patch_h * 14, patch_w * 14)),
T.CenterCrop((patch_h * 14, patch_w * 14)),
T.ToTensor(),
T.Normalize(mean=(0.485, 0.456, 0.406), std=(0.229, 0.224, 0.225)),
])
dinov2_vits14 = torch.hub.load('', 'dinov2_vits14',source='local').cuda()
features = torch.zeros(4, patch_h * patch_w, feat_dim)
imgs_tensor = torch.zeros(4, 3, patch_h * 14, patch_w * 14).cuda()
img_path = f'/kaggle/input/demo-image/1 (4).png'
img = Image.open(img_path).convert('RGB')
imgs_tensor[0] = transform(img)[:3]
with torch.no_grad():
features_dict = dinov2_vits14.forward_features(imgs_tensor)
features = features_dict['x_norm_patchtokens']
features = features.reshape(4 * patch_h * patch_w, feat_dim).cpu()
pca = PCA(n_components = 3)
pca.fit(features)
pca_features = pca.transform(features)
pca_features[:, 0] = (pca_features[:, 0] - pca_features[:, 0].min()) / (pca_features[:, 0].max() - pca_features[:, 0].min())
pca_features_fg = pca_features[:, 0] > 0.3
pca_features_bg = ~pca_features_fg
b = np.where(pca_features_bg)
pca.fit(features[pca_features_fg])
pca_features_rem = pca.transform(features[pca_features_fg])
for i in range(3):
pca_features_rem[:, i] = (pca_features_rem[:, i] - pca_features_rem[:, i].min()) / (pca_features_rem[:, i].max() - pca_features_rem[:, i].min())
pca_features_rgb = pca_features.copy()
pca_features_rgb[pca_features_fg] = pca_features_rem
pca_features_rgb[b] = 0
pca_features_rgb = pca_features_rgb.reshape(4, patch_h, patch_w, 3)
plt.imshow(pca_features_rgb[0][...,::-1])
plt.savefig('features_s14.png')
plt.show()
plt.close()
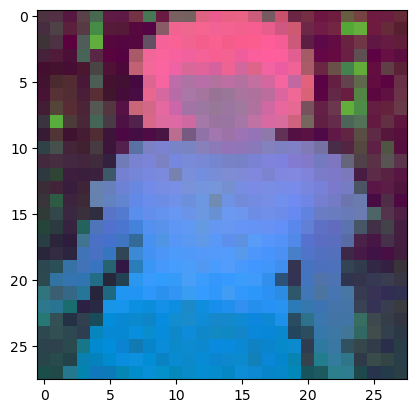
print('---s14---')
print(features)
print('---维度---')
print(features.shape)

print('---pca_features---')
print(pca_features)
print('---维度---')
print(pca_features.shape)
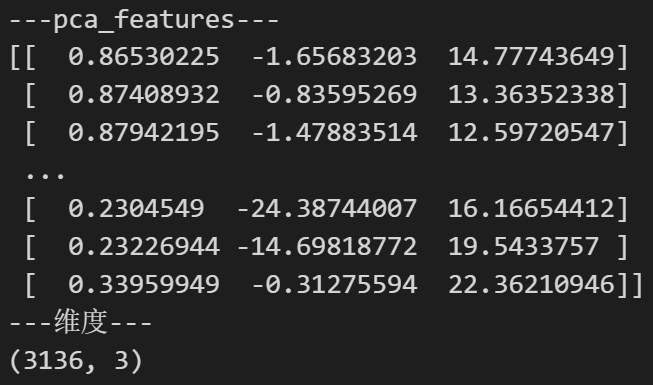
二、ViT-B/14
patch_h = 28
patch_w = 28
feat_dim = 768
transform = T.Compose([
T.GaussianBlur(9, sigma=(0.1, 2.0)),
T.Resize((patch_h * 14, patch_w * 14)),
T.CenterCrop((patch_h * 14, patch_w * 14)),
T.ToTensor(),
T.Normalize(mean=(0.485, 0.456, 0.406), std=(0.229, 0.224, 0.225)),
])
dinov2_vitb14 = torch.hub.load('', 'dinov2_vitb14',source='local').cuda()
features_b14 = torch.zeros(4, patch_h * patch_w, feat_dim)
imgs_tensor_b14 = torch.zeros(4, 3, patch_h * 14, patch_w * 14).cuda()
img_path = f'/kaggle/input/demo-image/1 (4).png'
img = Image.open(img_path).convert('RGB')
imgs_tensor_b14[0] = transform(img)[:3]
with torch.no_grad():
features_dict_b14 = dinov2_vitb14.forward_features(imgs_tensor_b14)
features_b14 = features_dict_b14['x_norm_patchtokens']
features_b14 = features_b14.reshape(4 * patch_h * patch_w, feat_dim).cpu()
pca = PCA(n_components = 3)
pca.fit(features_b14)
pca_features_b14 = pca.transform(features_b14)
pca_features_b14[:, 0] = (pca_features_b14[:, 0] - pca_features_b14[:, 0].min()) / (pca_features_b14[:, 0].max() - pca_features_b14[:, 0].min())
pca_features_fg_b14 = pca_features_b14[:, 0] > 0.3
pca_features_bg_b14 = ~pca_features_fg_b14
b = np.where(pca_features_bg_b14)
pca.fit(features_b14[pca_features_fg_b14])
pca_features_rem_b14 = pca.transform(features_b14[pca_features_fg_b14])
for i in range(3):
pca_features_rem_b14[:, i] = (pca_features_rem_b14[:, i] - pca_features_rem_b14[:, i].min()) \
/ (pca_features_rem_b14[:, i].max() - pca_features_rem_b14[:, i].min())
pca_features_rgb_b14 = pca_features_b14.copy()
pca_features_rgb_b14[pca_features_fg_b14] = pca_features_rem_b14
pca_features_rgb_b14[b] = 0
pca_features_rgb_b14 = pca_features_rgb_b14.reshape(4, patch_h, patch_w, 3)
plt.imshow(pca_features_rgb_b14[0][...,::-1])
plt.savefig('features_b14.png')
plt.show()
plt.close()
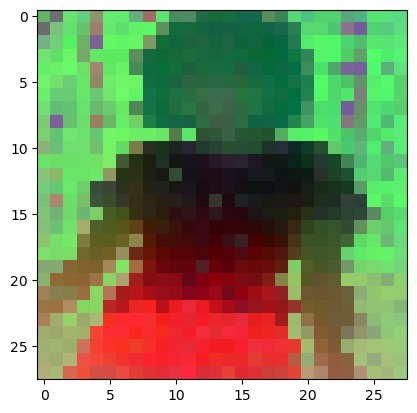
print('---b14---')
print(features_b14)
print('---维度---')
print(features_b14.shape)
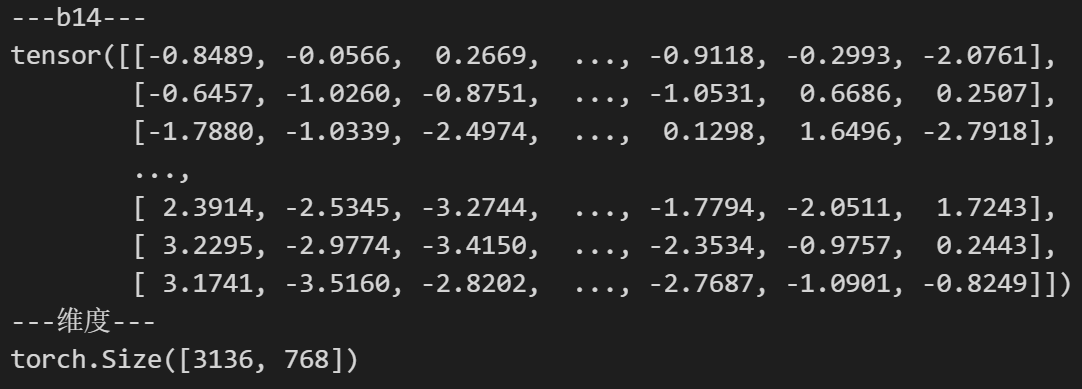
print('---pca_features_b14---')
print(pca_features_b14)
print('---维度---')
print(pca_features_b14.shape)

三、ViT-L/14
patch_h = 28
patch_w = 28
feat_dim = 1024
transform = T.Compose([
T.GaussianBlur(9, sigma=(0.1, 2.0)),
T.Resize((patch_h * 14, patch_w * 14)),
T.CenterCrop((patch_h * 14, patch_w * 14)),
T.ToTensor(),
T.Normalize(mean=(0.485, 0.456, 0.406), std=(0.229, 0.224, 0.225)),
])
dinov2_vitl14 = torch.hub.load('', 'dinov2_vitl14',source='local').cuda()
features_l14 = torch.zeros(4, patch_h * patch_w, feat_dim)
imgs_tensor_l14 = torch.zeros(4, 3, patch_h * 14, patch_w * 14).cuda()
img_path = f'/kaggle/input/demo-image/1 (4).png'
img = Image.open(img_path).convert('RGB')
imgs_tensor_l14[0] = transform(img)[:3]
with torch.no_grad():
features_dict_l14 = dinov2_vitl14.forward_features(imgs_tensor_l14)
features_l14 = features_dict_l14['x_norm_patchtokens']
features_l14 = features_l14.reshape(4 * patch_h * patch_w, feat_dim).cpu()
pca = PCA(n_components = 3)
pca.fit(features_l14)
pca_features_l14 = pca.transform(features_l14)
pca_features_l14[:, 0] = (pca_features_l14[:, 0] - pca_features_l14[:, 0].min()) \
/ (pca_features_l14[:, 0].max() - pca_features_l14[:, 0].min())
pca_features_fg_l14 = pca_features_l14[:, 0] > 0.3
pca_features_bg_l14 = ~pca_features_fg_l14
b = np.where(pca_features_bg_l14)
pca.fit(features_l14[pca_features_fg_l14])
pca_features_rem_l14 = pca.transform(features_l14[pca_features_fg_l14])
for i in range(3):
pca_features_rem_l14[:, i] = (pca_features_rem_l14[:, i] - pca_features_rem_l14[:, i].min()) \
/ (pca_features_rem_l14[:, i].max() - pca_features_rem_l14[:, i].min())
pca_features_rgb_l14 = pca_features_l14.copy()
pca_features_rgb_l14[pca_features_fg_l14] = pca_features_rem_l14
pca_features_rgb_l14[b] = 0
pca_features_rgb_l14 = pca_features_rgb_l14.reshape(4, patch_h, patch_w, 3)
plt.imshow(pca_features_rgb_l14[0][...,::-1])
plt.savefig('features_l14.png')
plt.show()
plt.close()

print('---l14---')
print(features_l14)
print('---维度---')
print(features_l14.shape)
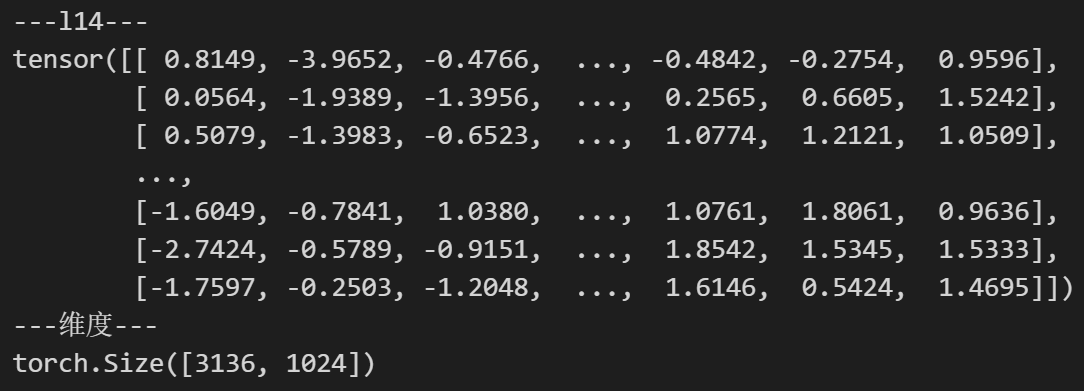
print('---pca_features_l14---')
print(pca_features_l14)
print('---维度---')
print(pca_features_l14.shape)
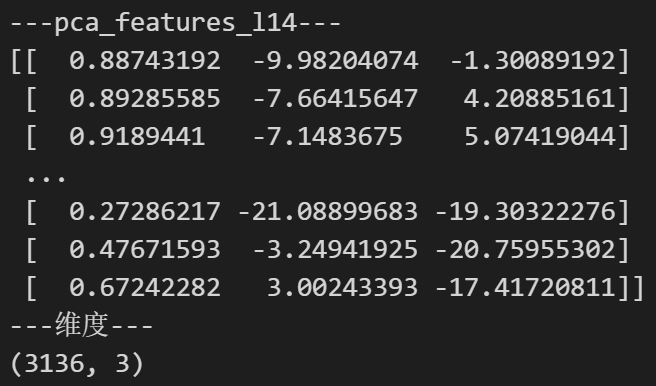
四、ViT-g/14
patch_h = 28
patch_w = 28
feat_dim = 1536
transform = T.Compose([
T.GaussianBlur(9, sigma=(0.1, 2.0)),
T.Resize((patch_h * 14, patch_w * 14)),
T.CenterCrop((patch_h * 14, patch_w * 14)),
T.ToTensor(),
T.Normalize(mean=(0.485, 0.456, 0.406), std=(0.229, 0.224, 0.225)),
])
dinov2_vitg14 = torch.hub.load('', 'dinov2_vitg14',source='local').cuda()
features_g14 = torch.zeros(4, patch_h * patch_w, feat_dim)
imgs_tensor_g14 = torch.zeros(4, 3, patch_h * 14, patch_w * 14).cuda()
img_path = f'/kaggle/input/demo-image/1 (4).png'
img = Image.open(img_path).convert('RGB')
imgs_tensor_g14[0] = transform(img)[:3]
with torch.no_grad():
features_dict_g14 = dinov2_vitg14.forward_features(imgs_tensor_g14)
features_g14 = features_dict_g14['x_norm_patchtokens']
features_g14 = features_g14.reshape(4 * patch_h * patch_w, feat_dim).cpu()
pca = PCA(n_components = 3)
pca.fit(features_g14)
pca_features_g14 = pca.transform(features_g14)
pca_features_g14[:, 0] = (pca_features_g14[:, 0] - pca_features_g14[:, 0].min()) \
/ (pca_features_g14[:, 0].max() - pca_features_g14[:, 0].min())
pca_features_fg_g14 = pca_features_g14[:, 0] > 0.3
pca_features_bg_g14 = ~pca_features_fg_g14
b = np.where(pca_features_bg_g14)
pca.fit(features_g14[pca_features_fg_g14])
pca_features_rem_g14 = pca.transform(features_g14[pca_features_fg_g14])
for i in range(3):
pca_features_rem_g14[:, i] = (pca_features_rem_g14[:, i] - pca_features_rem_g14[:, i].min()) \
/ (pca_features_rem_g14[:, i].max() - pca_features_rem_g14[:, i].min())
pca_features_rgb_g14 = pca_features_g14.copy()
pca_features_rgb_g14[pca_features_fg_g14] = pca_features_rem_g14
pca_features_rgb_g14[b] = 0
pca_features_rgb_g14 = pca_features_rgb_g14.reshape(4, patch_h, patch_w, 3)
plt.imshow(pca_features_rgb_g14[0][...,::-1])
plt.savefig('features_g14.png')
plt.show()
plt.close()
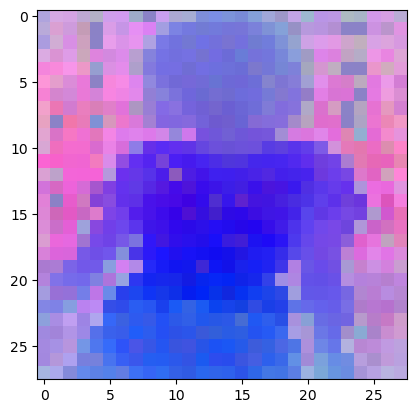
print('---g14---')
print(features_g14)
print('---维度---')
print(features_g14.shape)
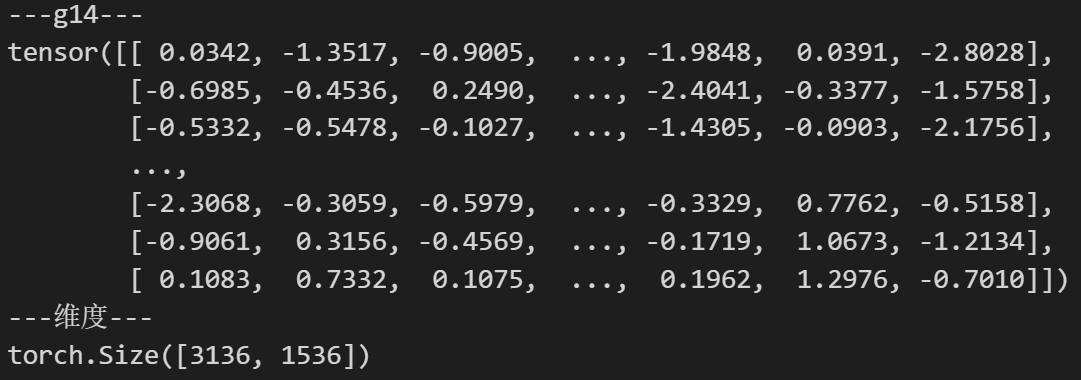
print('---pca_features_g14---')
print(pca_features_g14)
print('---维度---')
print(pca_features_g14.shape)
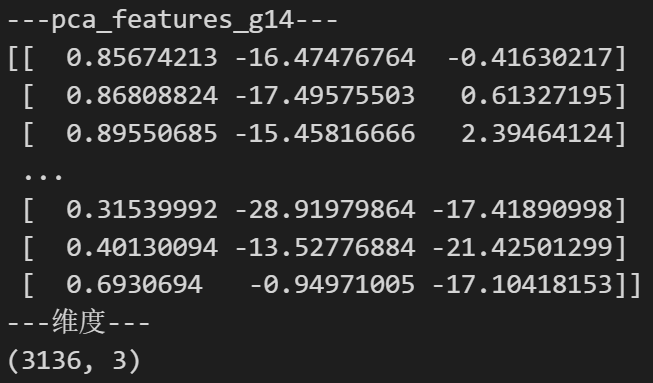