本文的运行环境是Win10,IDE是Pycharm,Python版本是3.6。
请先保证自己安装好Pycharm和Scrapy。
-
爬取的网站是国内著名的房天下网,网址:http://esf.xm.fang.com/,网站界面如下图所示。
网站列表界面.png
网站详情界面.png
可以看出该网站信息较为全面。 -
用Scrapy的Shell测试该网站是否能爬取。
方法是在任意位置打开cmd或者PowerShell,输入命令scrapy shell "esf.xm.fang.com",
一般来说不会出现错误,如果报错ImportError: DLL load failed: 操作系统无法运行 %1。,解决方法是把C:\Windows\System32目录下的libeay32.dll和ssleay32.dll删除即可。
确定命令正确后运行,结果如下图。测试能否爬取1.png
在In[1]:后输入命令view(response),确认命令正确后运行,会自动弹出浏览器窗口,如果出现如下图所示网站,则表示scrapy可以顺利从网站获取信息,即可以完成爬虫任务。
测试能够爬取2.png
从上图看出运行命令后打开的是本地的网站,即网站内容可以顺利从服务器缓存到本地。 -
在你的工程文件中按住Shit,鼠标右击呼唤出下图所示菜单。
选择下图所标识的在此处打开PowerShell窗口,cmd和PowerShell起到的效果相同。打开PowerShell.png
在PoweShell中运行命令scrapy startproject XiamenHouse
新建工程成功.png
新建工程成功后,在PowerShell中进入工程的文件,命令是 cd .\XiamenHouse
新建爬虫文件的命令是scrapy genspider house "esf.xm.fang.com"
新建爬虫成功.png -
用Pycharm打开爬虫工程
打开爬虫工程1.png选择工程所在的文件夹打开后,工程结构如下图所示。
image.png -
观察房屋详情界面,需要提取15个字段,分别是:标题title,总价price,首付downPayment,户型sizeType,建筑面积size,单价unitPrice,朝向orientation,楼层floor,装修decoration,社区community, 区域region,学校school,房源信息houseDetail,核心卖点keySellingPoint,小区配套equipment
月供是动态计算生成,较难爬取。image.png
image.png
根据上述字段总结,编写工程文件夹中的items.py文件
import scrapy
from scrapy import Field
class XiamenHouseItem(scrapy.Item):
title = Field()
price = Field()
downPayment = Field()
monthInstallment = Field()
sizeType = Field()
size = Field()
unitPrice = Field()
orientation = Field()
floor = Field()
decoration = Field()
community = Field()
region = Field()
school = Field()
houseDetail = Field()
keySellingPoint = Field()
equipment = Field()
- 编写工程文件夹中的house.py文件
需要进行多级页面爬取,从scrapy.http中引入Request方法。
爬虫名为house,用于scrapy crawl house命令中。
厦门市有6个区,分别为集美、翔安、同安、海沧、湖里、思明。
每个区有8个价格分类
价格分类.png
在start_urls这个列表中有6*8=48个url,parse函数用于解析这48个url,即分析每个区每个价格区间有多少页房价信息。
parse函数得到每个区每个价格区间的房价信息最大页面数之后,通过字符串拼接得到每一页的url。
每一页的url用yield Request(url,callback=self.parse1)发起请求,并调用parse1函数进行解析。
parse1函数用于获取每一页30个房价详情页面的url链接,通过yield Request(detailUrl,callback=self.parse2)发起请求,并调用parse2函数进行解析。
parse2的难点在于xpath的书写,需要懂xpath基本语法,书写时可以在浏览器的调试器中检查是否正确。
确定xpath书写正确,成功获取到字段后,将字段存入item,最后通过yield item交给管道处理。
python3可以把变量名设置为中文,但必须全部是中文,不能为100万以下这种形式。
# -*- coding: utf-8 -*-
import scrapy
from scrapy.http import Request
from XiamenHouse.items import XiamenHouseItem
import json
class HouseSpider(scrapy.Spider):
name = 'house'
allowed_domains = ['esf.xm.fang.com']
start_urls = []
region_dict = dict(
集美 = "house-a0354",
翔安 = "house-a0350",
同安 = "house-a0353",
海沧 = "house-a0355",
湖里 = "house-a0351",
思明 = "house-a0352"
)
price_dict = dict(
d100 = "d2100",
c100d200 = "c2100-d2200",
c200d250 = "c2200-d2250",
c250d300 = "c2250-d2300",
c300d400 = "c2300-d2400",
c400d500 = "c2400-d2500",
c500d600 = "c2500-d2600",
c600 = "c2600"
)
for region in list(region_dict.keys()):
for price in list(price_dict.keys()):
url = "http://esf.xm.fang.com/{}/{}/".format(region_dict[region],price_dict[price])
start_urls.append(url)
#start_urls共有48个,parse函数的作用是找出这48个分类中每个分类的最大页数
def parse(self, response):
pageNum = response.xpath("//span[@class='txt']/text()").extract()[0].strip('共').strip('页')
for i in range(1,int(pageNum)+1):
url = "{}-i3{}/".format(response.url.strip('/'),i)
yield Request(url,callback=self.parse1)
def parse1(self, response):
house_list = response.xpath("//div[@class='houseList']/dl")
for house in house_list:
if "list" in house.xpath("@id").extract()[0]:
detailUrl = "http://esf.xm.fang.com" + house.xpath("dd[1]/p/a/@href").extract()[0]
yield Request(detailUrl,callback=self.parse2)
def parse2(self, response):
def find(xpath,pNode=response):
if len(pNode.xpath(xpath)):
return pNode.xpath(xpath).extract()[0]
else:
return ''
item = XiamenHouseItem()
item['title'] = find("//h1[@class='title floatl']/text()").strip()
item['price'] = find("//div[@class='trl-item_top']/div[1]/i/text()") + "万"
item['downPayment'] = find("//div[@class='trl-item']/text()").strip().strip("首付约 ")
item['sizeType'] = find("//div[@class='tab-cont-right']/div[2]/div[1]/div[1]/text()").strip()
item['size'] = find("//div[@class='tab-cont-right']/div[2]/div[2]/div[1]/text()")
item['unitPrice'] = find("//div[@class='tab-cont-right']/div[2]/div[3]/div[1]/text()")
item['orientation'] = find("//div[@class='tab-cont-right']/div[3]/div[1]/div[1]/text()")
item['floor'] = find("//div[@class='tab-cont-right']/div[3]/div[2]/div[1]/text()") + ' ' + \
find("//div[@class='tab-cont-right']/div[3]/div[2]/div[2]/text()")
item['decoration'] = find("//div[@class='tab-cont-right']/div[3]/div[3]/div[1]/text()")
item['community'] = find("//div[@class='tab-cont-right']/div[4]/div[1]/div[2]/a/text()")
item['region'] = find("//div[@class='tab-cont-right']/div[4]/div[2]/div[2]/a[1]/text()").strip() + \
'-' + find("//div[@class='tab-cont-right']/div[4]/div[2]/div[2]/a[2]/text()").strip()
item['school'] = find("//div[@class='tab-cont-right']/div[4]/div[3]/div[2]/a[1]/text()")
detail_list = response.xpath("//div[@class='content-item fydes-item']/div[2]/div")
detail_dict = {}
for detail in detail_list:
key = find("span[1]/text()",detail)
value = find("span[2]/text()",detail).strip()
detail_dict[key] = value
item['houseDetail'] = json.dumps(detail_dict,ensure_ascii=False)
item['keySellingPoint'] = '\n'.join(response.xpath("//div[text()='核心卖点']/../div[2]/div/text()").extract()).strip()
item['equipment'] = '\n'.join(response.xpath("//div[text()='小区配套']/../div[2]/text()").extract()).strip()
yield item
- 编写工程文件夹中的pipelines.py文件
house_list用于收集每次传递进来的item
close_spider函数用于指明爬虫结束时进行的操作,函数中把house_list先转化为pandas的DataFrame,然后DataFrame转化为excel,最后通过time.process_time() 函数打印程序运行的总时间。
import time
import pandas as pd
class XiamenhousePipeline(object):
house_list = []
def process_item(self, item, spider):
self.house_list.append(dict(item))
return item
def close_spider(self, spider):
df = pd.DataFrame(self.house_list)
df.to_excel("厦门房价数据(房天下版).xlsx",columns=[k for k in self.house_list[0].keys()])
print("爬虫程序共运行{}秒".format(time.process_time()))
- 编写工程文件夹中settings.py文件
删除掉了文件中自带的注释内容,真正起作用的是下面这些代码。
BOT_NAME = 'XiamenHouse'
SPIDER_MODULES = ['XiamenHouse.spiders']
NEWSPIDER_MODULE = 'XiamenHouse.spiders'
USER_AGENT = 'Mozilla/5.0 (Windows NT 10.0; WOW64) AppleWebKit/537.36 (KHTML, like Gecko) Chrome/55.0.2883.87 Safari/537.36'
ROBOTSTXT_OBEY = False
CONCURRENT_REQUESTS = 96
ITEM_PIPELINES = {
'XiamenHouse.pipelines.XiamenhousePipeline': 300,
}
9.在工程文件夹下的任意一级目录在cmd或PowerShell中运行命令scrapy crawl house启动爬虫程序,运行程序产生的excel截图如下。
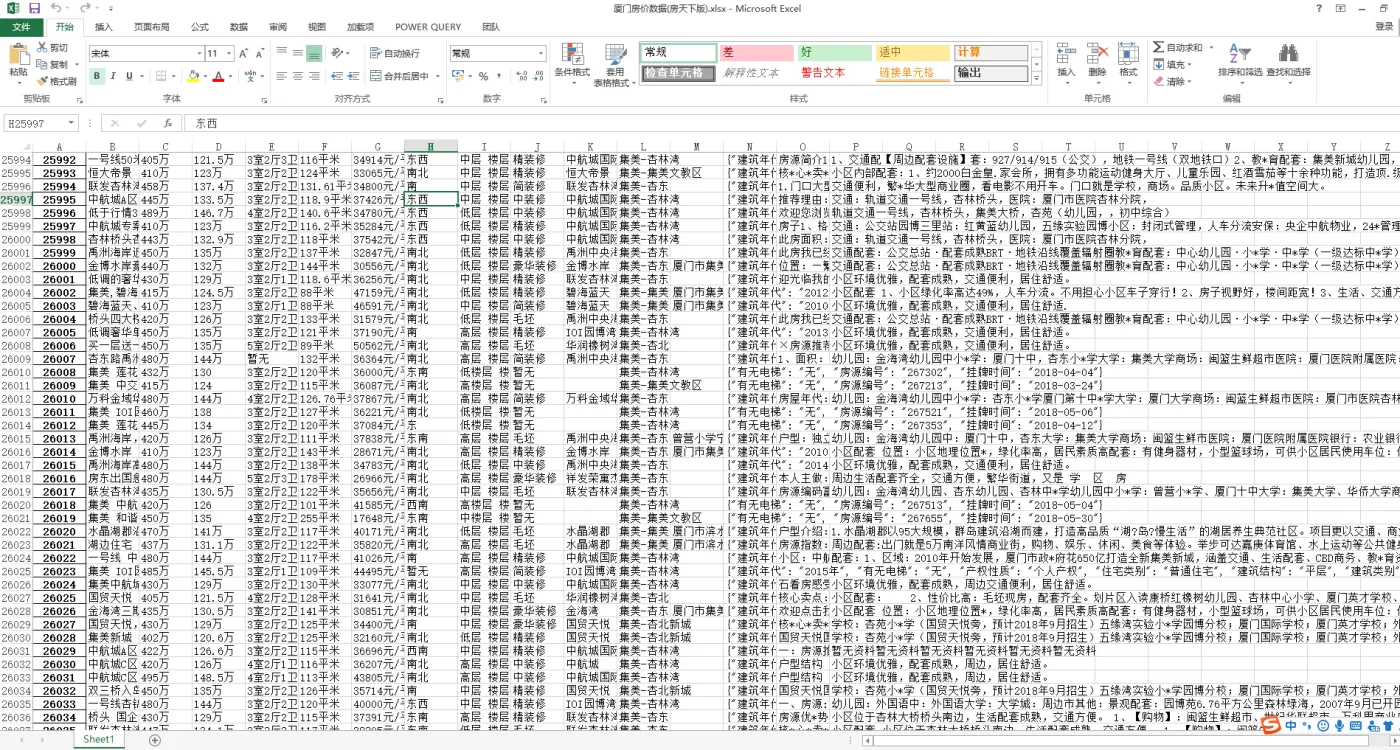
提示:
- 按照上述步骤正确进行,能够获取房天下网站厦门房产的全部信息,本文作者在2018年6月17日的测试结果是共爬取26332条房价信息,总共用时1363秒,即22分43秒。平均爬取速度为19.32条/秒,1159条/分。
- 确保程序能够正确运行,只需要完全复制上述4个文件即可,整个工程已经上传github,链接:
https://github.com/StevenLei2017/XiamenHouse - 自己编写代码,进行测试的时候,可以修改下面代码减少运行时间。
for region in list(region_dict.keys()):
for price in list(price_dict.keys()):
url = "http://esf.xm.fang.com/{}/{}/".format(region_dict[region],price_dict[price])
start_urls.append(url)
改为
for region in list(region_dict.keys())[:1]:
for price in list(price_dict.keys())[:1]:
url = "http://esf.xm.fang.com/{}/{}/".format(region_dict[region],price_dict[price])
start_urls.append(url)