写在前面:生成点云前提是已经安装好了libfreenect2和PCL,网上有许多这方面的大把教程,在这里就不多赘述了。
-->ubuntu16.04,pcl1.8<--
0,工件的点云展示
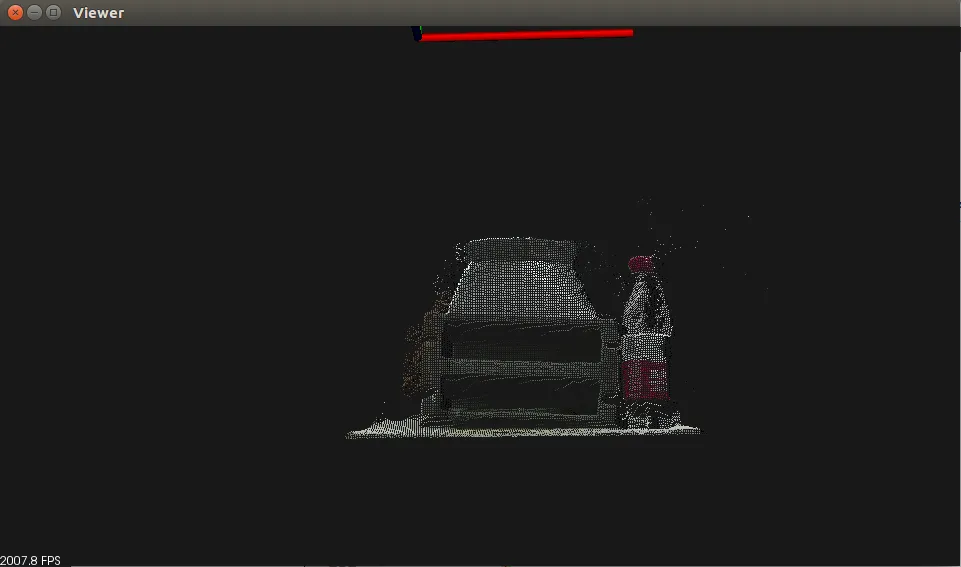
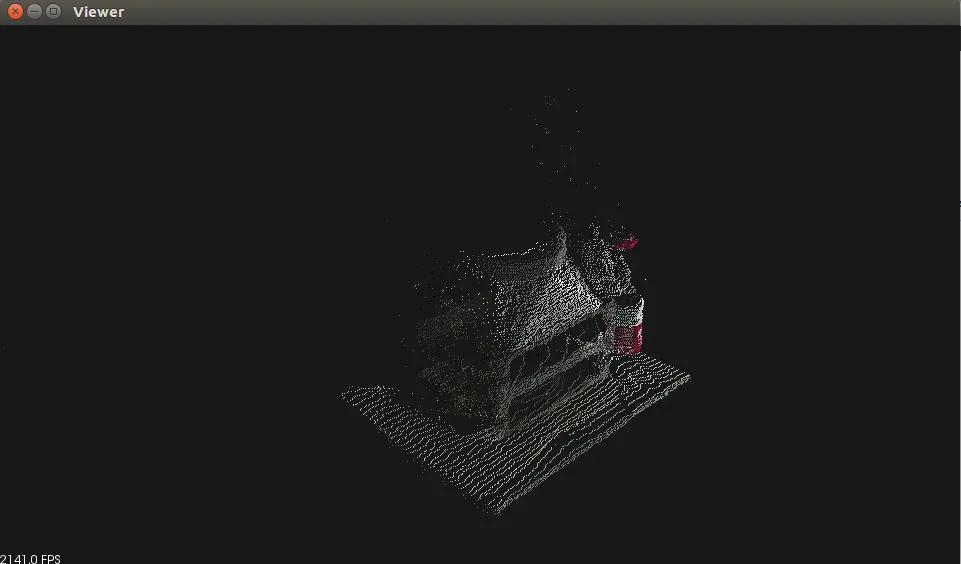
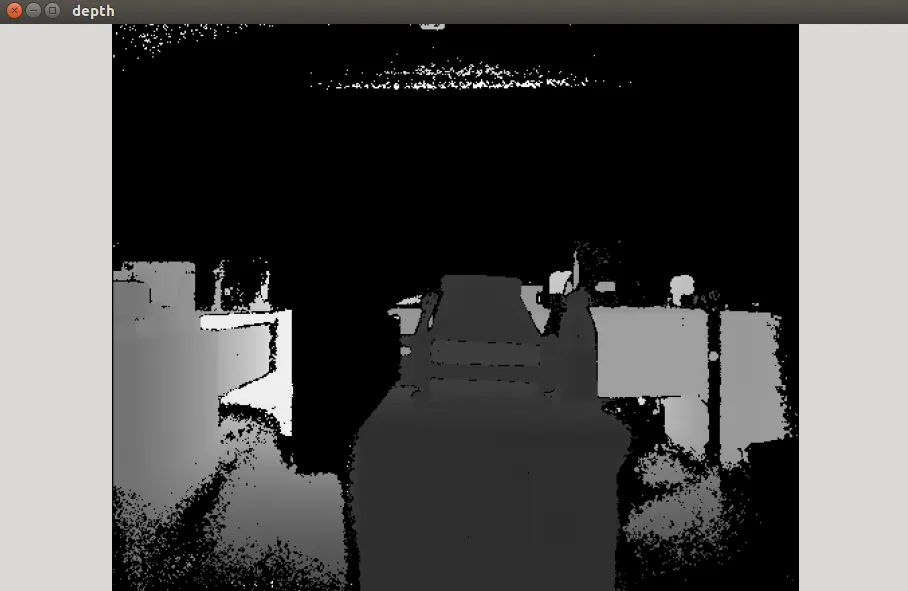
1,实现步骤
-
先通过libfreenect2提供的
getPointXYZRGB
函数,得到图像中每个像素点的空间坐标xyz以及rgb值。 运用点云库PCL中点云可视化功能,将获取到的每个点的xyz和rgb显示出来。
点云显示关键代码:
1,
pcl::visualization::CloudViewer viewer ("Viewer");
//创建一个显示点云的窗口,命名为Viewer。
2,PointCloud::Ptr cloud ( new PointCloud );
//使用智能指针,创建一个空点云。这种指针用完会自动释放。
这个空点云必须创建在while循环里面,否则最后显示出来的画面会无限叠加在窗口上,会变得很卡,之前写在了外面,找了好久才找到原来是这里写错了!
2,PointT p;
//定义一个用于储存坐标信息的点
3,cloud->points.push_back( p );
//将点P存入cloud
4,viewer.showCloud (cloud);
//将cloud可视化
注意:将下列两个文件复制到同一个文件夹中,并在终端中依次执行cmake .
,make
,便会生成一个可执行文件main
,输入./main
,就可以显示点云,如果是一片漆黑,是因为我在代码中设置来点云的范围,将if
语句去掉即可。
2,main.cpp
#include <iostream>
#include <stdio.h>
#include <iomanip>
#include <time.h>
#include <signal.h>
#include <opencv2/opencv.hpp>
#include <math.h>
#include <libfreenect2/libfreenect2.hpp>
#include <libfreenect2/frame_listener_impl.h>
#include <libfreenect2/registration.h>
#include <libfreenect2/packet_pipeline.h>
#include <libfreenect2/logger.h>
#include <pcl/io/pcd_io.h>
#include <pcl/point_types.h>
#include <pcl/visualization/cloud_viewer.h>
#include <pcl/visualization/pcl_visualizer.h>
#include <pcl/point_cloud.h>
using namespace std;
using namespace cv;
typedef pcl::PointXYZRGBA PointT;
typedef pcl::PointCloud<PointT> PointCloud;
enum
{
Processor_cl,
Processor_gl,
Processor_cpu
};
bool protonect_shutdown = false; // Whether the running application should shut down.
void sigint_handler(int s)
{
protonect_shutdown = true;
}
int main()
{
//定义变量
std::cout << "start!" << std::endl;
libfreenect2::Freenect2 freenect2;
libfreenect2::Freenect2Device *dev = 0;
libfreenect2::PacketPipeline *pipeline = 0;
//搜寻并初始化传感器
if(freenect2.enumerateDevices() == 0)
{
std::cout << "no device connected!" << std::endl;
return -1;
}
string serial = freenect2.getDefaultDeviceSerialNumber();
std::cout << "SERIAL: " << serial << std::endl;
//配置传输格式
#if 1 // sean
int depthProcessor = Processor_cl;
if(depthProcessor == Processor_cpu)
{
if(!pipeline)
//! [pipeline]
pipeline = new libfreenect2::CpuPacketPipeline();
//! [pipeline]
}
else if (depthProcessor == Processor_gl) // if support gl
{
#ifdef LIBFREENECT2_WITH_OPENGL_SUPPORT
if(!pipeline)
{
pipeline = new libfreenect2::OpenGLPacketPipeline();
}
#else
std::cout << "OpenGL pipeline is not supported!" << std::endl;
#endif
}
else if (depthProcessor == Processor_cl) // if support cl
{
#ifdef LIBFREENECT2_WITH_OPENCL_SUPPORT
if(!pipeline)
pipeline = new libfreenect2::OpenCLPacketPipeline();
#else
std::cout << "OpenCL pipeline is not supported!" << std::endl;
#endif
}
//启动设备
if(pipeline)
{
dev = freenect2.openDevice(serial, pipeline);
}
else
{
dev = freenect2.openDevice(serial);
}
if(dev == 0)
{
std::cout << "failure opening device!" << std::endl;
return -1;
}
signal(SIGINT, sigint_handler);
protonect_shutdown = false;
libfreenect2::SyncMultiFrameListener listener(
libfreenect2::Frame::Color |
libfreenect2::Frame::Depth |
libfreenect2::Frame::Ir);
libfreenect2::FrameMap frames;
dev->setColorFrameListener(&listener);
dev->setIrAndDepthFrameListener(&listener);
//启动数据传输
dev->start();
std::cout << "device serial: " << dev->getSerialNumber() << std::endl;
std::cout << "device firmware: " << dev->getFirmwareVersion() << std::endl;
//循环接收
libfreenect2::Registration* registration = new libfreenect2::Registration(dev->getIrCameraParams(), dev->getColorCameraParams());
libfreenect2::Frame undistorted(512, 424, 4), registered(512, 424, 4), depth2rgb(1920, 1080 + 2, 4);
Mat rgbmat, depthmat, rgbd, dst;
float x, y, z, color;
pcl::visualization::CloudViewer viewer ("Viewer"); //创建一个显示点云的窗口
while(!protonect_shutdown)
{
listener.waitForNewFrame(frames);
libfreenect2::Frame *rgb = frames[libfreenect2::Frame::Color];
libfreenect2::Frame *depth = frames[libfreenect2::Frame::Depth];
registration->apply(rgb, depth, &undistorted, ®istered, true, &depth2rgb);
PointCloud::Ptr cloud ( new PointCloud ); //使用智能指针,创建一个空点云。这种指针用完会自动释放。
for (int m = 0; m < 512 ; m++)
{
for (int n = 0 ; n < 424 ; n++)
{
PointT p;
registration->getPointXYZRGB(&undistorted, ®istered, n, m, x, y, z, color);
const uint8_t *c = reinterpret_cast<uint8_t*>(&color);
uint8_t b = c[0];
uint8_t g = c[1];
uint8_t r = c[2];
if (z<1.2 && y<0.2) //暂时先通过限定xyz来除去不需要的点,点云分割还在学习中。。。
{
p.z = -z;
p.x = x;
p.y = -y;
p.b = b;
p.g = g;
p.r = r;
}
cloud->points.push_back( p );
}
}
viewer.showCloud (cloud);
int key = cv::waitKey(1);
protonect_shutdown = protonect_shutdown || (key > 0 && ((key & 0xFF) == 27)); // shutdown on escape
listener.release(frames);
}
dev->stop();
dev->close();
delete registration;
#endif
std::cout << "stop!" << std::endl;
return 0;
}
3,CMakeLists.txt
cmake_minimum_required(VERSION 2.8)
project( main )
set(CMAKE_PREFIX_PATH ${CMAKE_PREFIX_PATH} $ENV{HOME}/freenect2/lib/cmake/freenect2)
find_package( OpenCV REQUIRED )
FIND_PACKAGE( PkgConfig REQUIRED )
FIND_PACKAGE( freenect2 REQUIRED )
find_package(PCL 1.2 REQUIRED)
include_directories("/usr/include/libusb-1.0/")
include_directories(${OpenCV_INCLUDE_DIRS})
include_directories( ${freenect2_INCLUDE_DIRS} )
include_directories(${PCL_INCLUDE_DIRS})
link_directories(${PCL_LIBRARY_DIRS})
add_definitions(${PCL_DEFINITIONS})
list(REMOVE_ITEM PCL_LIBRARIES "vtkproj4")
add_executable( main main.cpp )
target_link_libraries( main ${freenect2_LIBRARIES} ${OpenCV_LIBS} ${PCL_LIBRARIES} )