图论
无权图
交通运输,社交网络,互联网,工作的安排,闹区活动等等都可以用到图论处理。图可以分成两大类,一类是无向图,就是没有方向的,就好像两个人都互相认识一样,有向图就是单方面的联系,一个人认识另一个人,但是另一个人确不认识。当然,无向图也可以看成是一种特殊的有向图。图还可以根据权值分成两类,有权图和无权图,也就是边的权值,无权值只是表示了这个边存在与否而已,有权图表示的就是这个边的重要性,也可以看成是长度等等。图还有一个重要是性质,就是连通性的问题

简单图:不存在自环边和平行边的图。

图的表示方法有两种,图的核心就在于每一个点以及他们相连的边,通常我们就使用两种方法来表示, 邻接矩阵和邻接表。邻接矩阵就是用一个二维的矩阵来表示:


邻接表就是类似一个链表和数组的组合的数据结构了:

namespace Matrix {
class DenseGraph {
private:
int n, m;
bool directed;
vector<vector<bool>> g;
public:
DenseGraph(int n, bool directed) {
this->n = n;
this->m = 0;
this->directed = directed;
for (int i = 0; i < n; ++i) {
g.emplace_back(vector<bool>(n, false));
}
}
~DenseGraph() = default;
int V() {
return n;
}
int E() {
return m;
}
void addEdge(int v, int w) {
assert(v >= 0 && v < n);
assert(w >= 0 && w < n);
if (haveEdge(v, w)) {
return;
}
g[v][w] = true;
if (!this->directed) {
g[w][v] = true;
}
this->m++;
}
bool haveEdge(int v, int w) {
assert(v >= 0 && v < n);
assert(w >= 0 && w < n);
return g[v][w];
}
};
}
点数确定,边数先为0,等等从文件读进去。使用一个vector来存储,当然创建一个二维矩阵也可以。邻接表也是一样:
namespace list {
class SparseGraph {
private:
int n, m;
bool directed;
vector<vector<int>> g;
public:
SparseGraph(int n, bool directed) {
this->n = n;
this->directed = directed;
this->m = 0;
for (int i = 0; i < n; ++i) {
g.emplace_back(vector<int>());
}
}
~SparseGraph() = default;
int V() {
return n;
}
int E() {
return m;
}
void addEdge(int v, int w) {
assert(v >= 0 && v < n);
assert(w >= 0 && w < n);
if (haveEdge(v, w)) {
return;
}
g[v].emplace_back(w);
if (v != w && !this->directed) {
g[w].emplace_back(v);
}
this->m++;
}
bool haveEdge(int v, int w) {
assert(v >= 0 && v < n);
assert(w >= 0 && w < n);
for (auto var : g[v]) {
if (var == w) {
return true;
}
}
return false;
}
};
}
广度深度遍历
接下来就是图比较重要的操作,遍历操作,通过一个点来遍历相邻的邻边。通常比较常用的方法就是遍历操作,遍历每一个点,矩阵就看看是不是true,表就直接打印即可。但是这样在后面的广度遍历和深度遍历那么邻接表和邻接矩阵就要写两遍了,所以这里使用一个迭代器来操作,迭代器当做一个借口,返回当前这个点所连接的点是什么。
//interator
class adjIterator {
private:
SparseGraph &G;
int v;
int index;
public:
adjIterator(SparseGraph &graph, int v) : G(graph) {
assert(v < graph.n);
this->v = v;
this->index = 0;
}
int begin(){
if (!G.g[v].empty()){
return G.g[v][this->index];
}
return -1;
}
int next(){
index ++;
if (index < G.g[v].size()){
return G.g[v][index];
}
return -1;
}
bool end(){
return index >= G.g[v].size();
}
};
写成一个类,index来指示当前遍历到哪里了。这里的构造函数由于初始化的参数是一个引用变量,所以需要列表初始化,因为引用变量的初始化一定要列表初始化才可以。begin得到第一个元素,next下一个,end判断是否结束,和for三连是一样的。

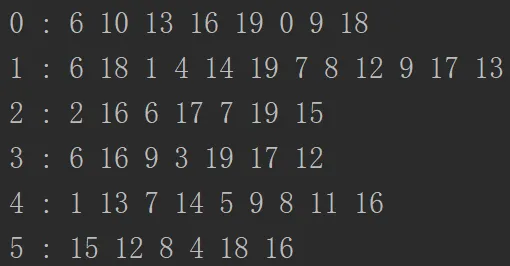
对于邻接矩阵的遍历有些许不同:
class adjIterator{
private:
DenseGraph &G;
int v;
int index;
public:
adjIterator(DenseGraph &graph, int v): G(graph){
this->v = v;
this->index = -1;
}
int begin(){
index = -1;
return next();
}
int next(){
for (index += 1; index < G.V(); index ++){
if (G.g[v][index]){
return index;
}
}
return -1;
}
bool end(){
return index >= G.V();
}
};
因为这个时候是遍历所有的点,是true的就输出,不是的跳过,所以这个时候begin第一个输出的就是第一个为treu的点而不是index为0的点了。所以设成-1,通过next来找第一个为true的点即可,之后其他的同样。现在添加一个工具类,从文件读入一个图:

第一行是节点数和边数,接下来就是边的两个端点。
template <typename Graph>
class ReadGraph{
public:
ReadGraph(Graph &graph, const string &filename){
ifstream file(filename);
string line;
int V, E;
assert(file.is_open());
assert(getline(file, line));
stringstream ss(line);
ss >> V >> E;
assert(V == graph.V());
for (int i = 0; i < E; ++i) {
assert(getline(file, line));
stringstream ss(line);
int a, b;
ss >> a >> b;
graph.addEdge(a, b);
}
}
};
使用模板类,是为了可以把邻接矩阵和邻接表都读进来。接下来的操作都很简单了。接下来就是图比较重要的操作了,图的遍历了。图的操作分为两种广度优先遍历,深度优先遍历。首先是深度遍历,就是从一个节点开始遍历到不能再遍历为止,图和树不一样,图是存在了环的,如果遍历过了那就必须设置已读的标记。
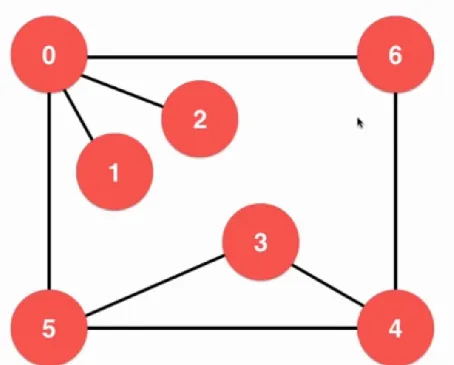
比如从0开始,首先第一个是0,之后就是1,2,因为这两个节点是没有连接到其他节点的,之后就是5,5连接了034,但是0已经看过了,就遍历3,3遍历4之后回来,最后就剩下和3节点相连的6了。从深度优先遍历也可以看出这个图的连通分量是多少。如果一直都是深度没有断开过,那肯定就是1了。这个时候就体现出了迭代器的好处,很好的屏蔽了底层数据结构的区别,直接复用即可。深度优先搜索还有一个性质,可以求解联通分量。如果深度一次完了之后还没有被遍历到的点那么就不属于这个联通分量了。同时也可以求解两个点是不是连在一起的,在同一个联通分量的那么肯定就是相连的了,总体来看,还是和连通分量有关系。
using namespace std;
template<typename Graph>
class Component {
private:
int *id;
Graph &G;
bool *visited;
int Ccount;
void dfs(int v) {
visited[v] = true;
id[v] = Ccount;
cout << v << " ";
typename Graph::adjIterator adj(G, v);
for (int i = adj.begin(); !adj.end(); i = adj.next()) {
if (!visited[i]){
dfs(i);
}
}
}
public:
Component(Graph &graph) : G(graph) {
visited = new bool[G.V()];
id = new int[G.V()];
Ccount = 0;
for (int i = 0; i < G.V(); ++i) {
visited[i] = false;
id[i] = -1;
}
for (int i = 0; i < G.V(); ++i) {
if (!visited[i]) {
dfs(i);
Ccount++;
}
}
}
int count(){
return Ccount;
}
bool isConnected(int v, int w){
return id[v] == id[w];
}
~Component() {
delete [] visited;
}
};
count用来计算连通分量的个数,id用来计算这些点是属于哪一个连通分量的,遍历这个点的所有点,这里就用迭代器很好的屏蔽了不同数据结构实现的区别,如果没有访问过那就从这个点开始深度优先,然后递归下去。既然id就是连通分量的代号,那么直接等于count即可。判断是不是在一个连通分量里面的就直接对比id即可。

深度优先还有一个很重要的应用,就是寻路。这里的寻路只是找到一条路而已,没有说是最短路,事实上很多时候都是随机路径,因为有时候遍历的顺序不一样,得到的路径也是不一样的。
template<typename Graph>
class Path {
private:
bool *visited;
int *from;
Graph &G;
int s;
void dfs(int v) {
visited[v] = true;
typename Graph::adjIterator adj(G, v);
for (int i = adj.begin(); !adj.end(); i = adj.next()) {
if (!visited[i]) {
from[i] = v;
dfs(i);
}
}
}
public:
Path(Graph graph, int s) : G(graph) {
assert(s >= 0 && s < G.V());
visited = new bool[G.V()];
from = new int[G.V()];
for (int i = 0; i < G.V(); ++i) {
visited[i] = false;
from[i] = -1;
}
this->s = s;
dfs(s);
}
~Path() {
delete[] from;
delete[] visited;
}
void path(int w, vector<int> &vec) {
stack<int> s;
int p = w;
while (p != -1) {
s.push(p);
p = from[p];
}
vec.clear();
while (!s.empty()) {
vec.push_back(s.top());
s.pop();
}
}
bool hasPath(int w) {
assert(w >= 0 && w < G.V());
return visited[w];
}
void showPath(int w) {
for (int i = 0; i < G.V(); ++i) {
cout << visited[i] << " ";
}
vector<int> vec;
path(w, vec);
for (auto v: vec) {
cout << v << " ";
}
cout << endl;
}
};
visited查看这些节点有没有被访问过,from查看这个节点是哪里来的,DFS遍历如果这个节点是没有被访问过的,那就赋值看看他是从哪个节点过来的,最后显示即可。最后需要反向查找。

图的广度优先遍历也需要用到队列。首先把这个节点周围的放入队列,如果队列不为空,那就直接出来一个把出来的那个周围的节点也塞进去,以此类推。广度优先遍历在图里面也叫层序遍历,一层一层的来,所以,先遍历到的肯定比后遍历到的距离原点要短,所以如果这个图是无权图,是可以使用这种方法来找到最短路径。每一层都加上1即可。
template <typename Graph>
class bfs{
private:
Graph &G;
int s;
bool *visited;
int *from;
int *ord;
public:
bfs(Graph &graph, int s): G(graph){
assert(s >= 0 && s < graph.V());
from = new int[graph.V()];
ord = new int[graph.V()];
visited = new bool[graph.V()];
for (int i = 0; i < graph.V(); ++i) {
visited[i] = false;
from[i] = -1;
ord[i] = -1;
}
this->s = s;
queue<int> q;
q.push(s);
ord[s] = 0;
visited[s] = true;
while (!q.empty()){
int w = q.front();
cout << w << " ";
q.pop();
typename Graph::adjIterator adj(graph, w);
for (int i = adj.begin(); !adj.end(); i = adj.next()) {
if (!visited[i] ){
q.push(i);
visited[i] = true;
from[i] = w;
ord[i] = ord[w] + 1;
}
}
}
}
void showShortPath(int w){
stack<int> s;
if (visited[w]){
int p = w;
while (p != -1){
s.push(p);
p = from[p];
}
}
vector<int> vec;
while (!s.empty()){
vec.push_back(s.top());
s.pop();
}
for (auto v: vec) {
cout << v << " ";
}
cout << endl;
}
};
from就是存储上一个节点,ord存储距离起始点的距离是多少。

BFS找到的就是最短路径。DFS其实也可以找到最短路径,但是是随机的,它和你存储图的顺序有不同,和图的结构也有关系,但是BFS是一定的,而且BFS的最短路径是不止一条。
带权图
上面所提到的都是无权图,就是仅仅是一条边而已,但是有权图每一条边都会带有一个权值,这个权值的意义可能是这条路的长度,平坦程度等等。上面的无权图有两种存储方式,那么自然有权图也有两种了,通过无权图变换过来。
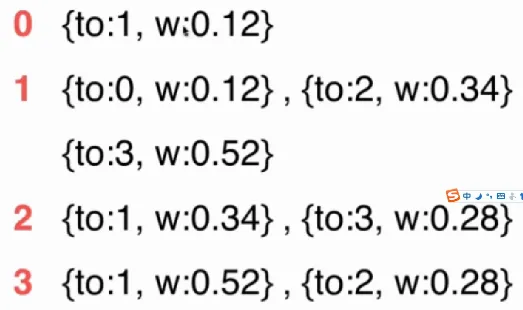
class Edge {
private:
int a, b;
Weight weight;
public:
Edge() {}
Edge(int a, int b, Weight weight) {
this->a = a;
this->b = b;
this->weight = weight;
}
~Edge() = default;
Weight wt(){
return weight;
}
int Other(int v) {
assert(v == a || v == b);
return v == a ? b : a;
}
friend ostream& operator<<(ostream &os, const Edge &e){
os << e.a << "->" << e.b << " : " << e.weight;
return os;
}
bool operator<(Edge<Weight>& e){
return weight < e.wt();
}
bool operator<=(Edge<Weight>& e){
return weight <= e.wt();
}
bool operator>(Edge<Weight>& e){
return weight < e.wt();
}
bool operator>=(Edge<Weight>& e){
return weight < e.wt();
}
bool operator==(Edge<Weight>& e){
return weight < e.wt();
}
};
Edge类存储了起始点和终点和权值。重载了一些比较和输出符号,等一下的输出和比较都要用到。邻接矩阵需要修改的不多:
using namespace std;
namespace Span {
template<typename Weight>
class DenseGraph {
private:
int n, m;
bool directed;
vector<vector<Edge<Weight> *>> g;
public:
DenseGraph(int n, bool directed) {
this->n = n;
this->m = 0;
this->directed = directed;
for (int i = 0; i < n; ++i) {
g.emplace_back(vector<Edge<Weight> *>(n, NULL));
}
}
~DenseGraph() {
for (int i = 0; i < n; ++i) {
for (int j = 0; j < n; ++j) {
if (g[i][j] != NULL) {
delete g[i][j];
}
}
}
}
int V() {
return n;
}
int E() {
return m;
}
void addEdge(int v, int w, Weight weight) {
assert(v >= 0 && v < n);
assert(w >= 0 && w < n);
if (haveEdge(v, w)) {
delete g[v][w];
if (!this->directed) {
delete g[w][v];
}
m--;
}
g[v][w] = new Edge<Weight>(v, w, weight);
if (!this->directed) {
g[w][v] = new Edge<Weight>(w, v, weight);
}
this->m++;
}
bool haveEdge(int v, int w) {
assert(v >= 0 && v < n);
assert(w >= 0 && w < n);
return g[v][w] != NULL;
}
void show() {
for (int i = 0; i < g.size(); ++i) {
for (int j = 0; j < g[i].size(); ++j) {
if (g[i][j] != NULL){
cout << g[i][j]->wt() << " ";
} else{
cout << "NULL" << " ";
}
}
cout << endl;
}
}
class adjIterator {
private:
DenseGraph &G;
int v;
int index;
public:
adjIterator(DenseGraph &graph, int v) : G(graph) {
this->v = v;
this->index = -1;
}
Edge<Weight> begin() {
index = -1;
return next();
}
Edge<Weight> next() {
for (index += 1; index < G.V(); index++) {
if (G.g[v][index]) {
return G.g[v][index];
}
}
return NULL;
}
bool end() {
return index >= G.V();
}
};
};
}
邻接表也是一样。
using namespace std;
namespace Sparse {
template<typename Weight>
class SparseGraph {
private:
int n, m;
bool directed;
vector<vector<Edge<Weight> *>> g;
public:
void show() {
for (int i = 0; i < n; ++i) {
for (int j = 0; j < g[i].size(); ++j) {
cout << g[i][j]->wt() << " ";
}
cout << endl;
}
}
SparseGraph(int n, bool directed) {
this->n = n;
this->directed = directed;
this->m = 0;
for (int i = 0; i < n; ++i) {
g.emplace_back(vector<Edge<Weight> *>());
}
}
~SparseGraph() {
for (int i = 0; i < n; ++i) {
for (int j = 0; j < g[i].size(); ++j) {
delete g[i][j];
}
}
}
int V() {
return n;
}
int E() {
return m;
}
void addEdge(int v, int w, Weight weight) {
assert(v >= 0 && v < n);
assert(w >= 0 && w < n);
if (haveEdge(v, w)) {
return;
}
g[v].emplace_back(new Edge<Weight>(v, w, weight));
if (v != w && !this->directed) {
g[w].emplace_back(new Edge<Weight>(w, v, weight));
}
this->m++;
}
bool haveEdge(int v, int w) {
assert(v >= 0 && v < n);
assert(w >= 0 && w < n);
for (auto var : g[v]) {
if (var->Other(v) == w) {
return true;
}
}
return false;
}
//interator
class adjIterator {
private:
SparseGraph &G;
int v;
int index;
public:
adjIterator(SparseGraph &graph, int v) : G(graph) {
assert(v < graph.n);
this->v = v;
this->index = 0;
}
int begin() {
if (!G.g[v].empty()) {
return G.g[v][this->index];
}
return -1;
}
int next() {
index++;
if (index < G.g[v].size()) {
return G.g[v][index];
}
return -1;
}
bool end() {
return index >= G.g[v].size();
}
};
};
}
修改一下show函数即可。
最小生成树
要讨论的第一个有权图问题就是最小生成树问题。对于一个完全连通的一个带权图能否找到这个图属于的一个最小生成树,这个生成树要连接所有的顶点,并且不能有环,因为树就没有环,而判断有没有环就可以用并查集来判断了。如果这棵树所有的权值相加都是最小的那么就叫做是最小生成树。电缆的布线问题就用到这些。最小生成树一般针对带权的无向图,并且需要连通,不连通怎么都到不了所有的节点。连通所有的点就需要找V-1条边,连接V个顶点。
首先要介绍一个切分定理。把图中的节点切分成两部分,成为一个切分。

Prim Algorithm
prim算法就是根据这个思想来完成最小生成树的构建。首先一开始所有点都是同一个阵营,首先遍历第一个点,也就是第0号点,那么这个点就出了这个阵营,把它相邻的边都扔进一个最小堆进行维护,如果当前的堆不是空的,那么就出第一个最小的边,但是出的时候需要判断这个边的两个顶点是不是不同阵营的,因为在遍历的过程中每一个点的阵营的改变的全局的,会影响到其他边本来的状态,所以取出来的时候需要判断一下,然后从取出来处理的那条边做突破口,看它两边的那个点是没有被访问的,继续上述步骤。准备一个marked boolean数组,false一边true一边,true表示被访问了,也就是被访问的一边没有被访问的一边。
namespace MinimumSpanTree_Prim {
template<typename Graph, typename Weight>
class Prim {
private:
Graph &G;
MinHeap<Edge<Weight>> pq;
bool *marked;
vector<Edge<Weight>> MinimumEdge;
Weight mstWeight;
void visit(int v){
assert( !marked[v] );
marked[v] = true;
typename Graph::adjIterator adj(G, v);
for (Edge<Weight> *e = adj.begin(); !adj.end() ; e = adj.next()) {
if (!marked[e->Other(v)]){
cout << e->wt() << " ";
pq.insert(*e);
}
}
cout << endl;
pq.show();
}
public:
Prim(Graph &graph) : G(graph), pq(MinHeap<Edge<Weight>>(G.E())){
marked = new bool[ G.V() ];
for (int i = 0; i < G.V(); ++i) {
marked[i] = false;
}
MinimumEdge.clear();
visit(0);
while ( !pq.isEmpty() ){
Edge<Weight> e = pq.extractMin();
cout << e.wt() << endl;
if (marked[e.v()] == marked[e.w()]){
continue;
}
MinimumEdge.push_back(e);
if ( !marked[e.v()] ){
visit(e.v());
} else{
visit(e.w());
}
}
mstWeight = MinimumEdge[0].wt();
for (int j = 1; j < MinimumEdge.size(); ++j) {
mstWeight += MinimumEdge[j].wt();
}
}
~Prim(){
delete [] marked;
}
vector<Edge<Weight>> mstEdges(){
return MinimumEdge;
}
Weight result(){
return mstWeight;
}
};
}
访问这个节点的时候就遍历这个节点的所有边,塞进堆里面动态维护。
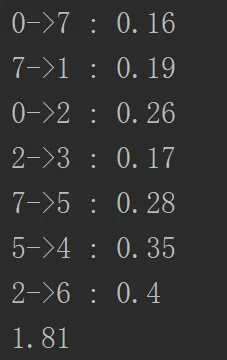
当最小堆不为空,那么就继续,所有while这个循环的复杂度就是E,也就是边数,而出堆的复杂度是
Kruskal Algorithm
在寻找最短的一条边的时候,会不会每一次寻找最短的一条边都会是属于最小生成树的呢?其实就是这样,因为我们找到一个最短边之后就把这条最小边的一个点看做是另一个阵营,这样每一次我们只需要防止形成环就好了。首先先对所有的边进行排序,每一次取一条,只要不形成环即可。那么问题来了,怎么判断是不是环?之前提到了并查集,并查集就可以判断是不是形成环。
namespace MinimumSpanTree_Kruskal{
template <typename Graph, typename Weight>
class Kruskal{
private:
vector<Edge<Weight>> mst;
Weight mstWeight;
public:
Kruskal(Graph &graph){
MinHeap<Edge<Weight>> pg(graph.E());
for (int i = 0; i < graph.V(); ++i) {
typename Graph :: adjIterator adj(graph, i);
for (Edge<Weight> *e = adj.begin(); !adj.end() ; e = adj.next()) {
if (e->v() < e->w()){
pg.insert(*e);
}
}
}
UF_version3::unionFind unionFind(graph.E());
while (!pg.isEmpty() && mst.size() < graph.V() - 1){
Edge<Weight> e = pg.extractMin();
if (unionFind.isConnected(e.v(), e.w())){
continue;
}
mst.push_back(e);
unionFind.unionElements(e.v(), e.w());
}
mstWeight =mst[0].wt();
for (int j = 1; j < mst.size(); ++j) {
mstWeight += mst[j].wt();
}
}
vector<Edge<Weight>> mstEdges(){
return mst;
}
Weight result(){
return mstWeight;
}
};
}
思路和实现都很简单,不用多逼逼。
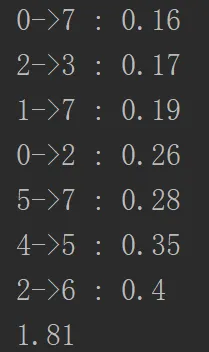
Summary

最短路径问题
最短路径问题理论上是对于有向图的,但是无向图本质上也是一种特殊的有向图,所以这里所写的最短路径对于无向图也是有效的。之前在无权图的时候是使用是广度遍历找到当前点到所有点的最短路径,而加上了权值之后不能单单从一次广度就判断那条边的最小的,因为权值的叠加可能更会使得最下的权值空前的增大。所以每一次都要在当前的节点判断一下,绕一下过后是不是比原来直接到达目的地的还要短,这个操作就叫做松弛操作。
dijkstra算法
使用dijkstra算法又前提条件,这个算法的权值是不能有负权值,算法的复杂度是的,最小生成树Prim算法的改进也是这个复杂度。用一个最简单的图:


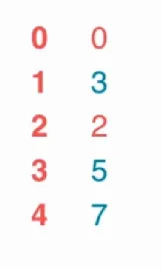
template <typename Graph, typename Weight>
class Dijkstra{
private:
Graph &G;
int s;
Weight *distTo;
bool *marked;
vector<Edge<Weight> *> from;
public:
Dijkstra(Graph &graph, int s) : G(graph){
this->s = s;
distTo = new Weight[G.V()];
marked = new bool[G.V()];
for (int i = 0; i < G.V(); ++i) {
distTo[i] = Weight();
marked[i] = false;
from.push_back(NULL);
}
IndexMinHeap<Weight> pq(G.V());
distTo[s] = Weight();
marked[s] = true;
pq.insert(s, distTo[s]);
while (!pq.isEmpty()){
int v = pq.extractMinIndex();
marked[v] = true;
typename Graph :: adjIterator adj(G, v);
for (auto e = adj.begin(); !adj.end(); e = adj.next()) {
int w = e->Other(v);
if (!marked[w]){
if (from[w] == NULL || distTo[v] + e->wt() < distTo[w] ){
distTo[w] = distTo[v] + e->wt();
from[w] = e;
if (pq.contain(w)){
pq.change(w, distTo[w]);
} else{
pq.insert(w, distTo[w]);
}
}
}
}
}
}
void show(){
stack<Edge<Weight> *> ss;
for (int i = 1; i < G.V(); ++i) {
vector<Edge<Weight>> vec;
cout << i << " :" << endl;
Edge<Weight> * e = from[i];
while (e->v() != this->s){
ss.push(e);
e = from[e->v()];
}
ss.push(e);
while( !ss.empty() ){
e = ss.top();
vec.push_back( *e );
ss.pop();
}
for (int j = 0; j < vec.size(); ++j) {
cout << vec[j].v() << " ";
if( j == vec.size()-1 )
cout<<vec[j].w()<<endl;
}
//cout << distTo[i] << endl;
}
}
~Dijkstra(){
delete [] distTo;
delete [] marked;
}
};
s就是开始遍历的节点,首先起始点肯定是要被访问的,所以自然marked就是true了,代表被访问过,起始点的距离直接就是0,因为起始点是到自己没有路径。压进堆里面,如果堆不为空,遍历最小边的原点,这个时候就是属于松弛的过程了,看看有没有过当前最小的节点可以得到更小的边,如果有,那么看看当前堆里面有没有包含了节点的最小路径,包含了就替换。

Bellman-Ford算法
上面提到的Dijskra算法是不可以处理负权边的,因为每一次找到最小的权值就判定它就是最短路径中的一条了,从已经的最小的路径再做松弛操作,也就是绕回来只能更大。但是如果是存在了负权边:
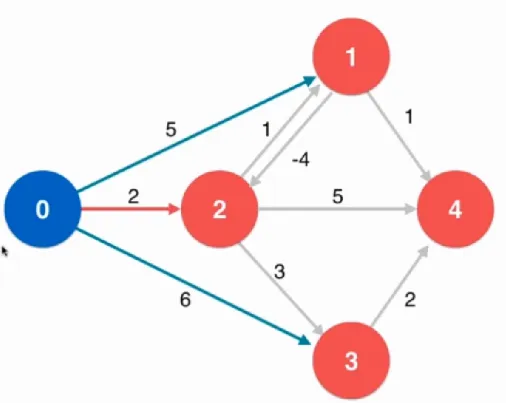

Bellman-Ford算法的前提就是不能有负权环,但是这个前提不是一定的条件,因为运行的时候是可以知道有没有负权环,如果有那么是出不来结果的。算法复杂度
实现起来其实很简单:
namespace Ford{
template <typename Graph, typename Weight>
class BellmanFord{
private:
Graph &G;
int s;
Weight* distTo;
vector<Edge<Weight>*> from;
bool hasNegativeCycle;
public:
bool detectNegativeCycle(){
for (int i = 0; i < G.V(); ++i) {
typename Graph::adjIterator adj(G, i);
for (auto e = adj.begin(); !adj.end() ; e = adj.next()) {
if (!from[e->w()] || distTo[e->v()] + e->wt() < distTo[e->w()]){
return true;
}
}
}
return false;
}
BellmanFord(Graph &graph, int s):G(graph){
this->s = s;
distTo = new Weight[G.V()];
for (int i = 0; i < G.V(); ++i) {
from.push_back(NULL);
}
distTo[s] = Weight();
for (int pass = 1; pass < G.V(); ++pass) {
for (int i = 0; i < G.V(); ++i) {
typename Graph::adjIterator adj(G, i);
for (auto e = adj.begin(); !adj.end() ; e = adj.next()) {
if (!from[e->w()] || distTo[e->v()] + e->wt() < distTo[e->w()]){
distTo[e->w()] = distTo[e->v()] + e->wt();
from[e->w()] = e;
}
}
}
}
hasNegativeCycle = detectNegativeCycle();
}
void show(){
stack<Edge<Weight> *> ss;
for (int i = 1; i < G.V(); ++i) {
vector<Edge<Weight>> vec;
cout << i << " :" << endl;
Edge<Weight> * e = from[i];
while (e->v() != this->s){
ss.push(e);
e = from[e->v()];
}
ss.push(e);
while( !ss.empty() ){
e = ss.top();
vec.push_back( *e );
ss.pop();
}
for (int j = 0; j < vec.size(); ++j) {
cout << vec[j].v() << " ";
if( j == vec.size()-1 )
cout<<vec[j].w()<<endl;
}
//cout << distTo[i] << endl;
}
}
};
}
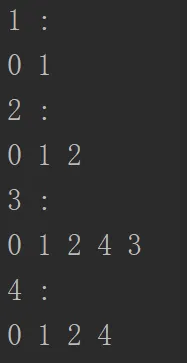
做完V-1次松弛操作之后,再做一次看看能不能找到更小的,如果还可以,那么就证明是有环了。
最后附上github地址:https://github.com/GreenArrow2017/DataStructure/tree/master/DataStucture