1. 客户端接口 Client interface
为了展示一个RPC服务是如何使用的,我们将创建一段很简单的客户端class。 它将会向外提供名字为call的函数,这个call会发送RPC请求并且阻塞知道收到RPC运算的结果。代码如下:
fibonacci_rpc = FibonacciRpcClient() result = fibonacci_rpc.call(4) print "fib(4) is %r" % (result,)
2. 回调函数队列 Callback queue
总体来说,在RabbitMQ进行RPC远程调用是比较容易的。client发送请求的Message然后server返回响应结果。为了收到响应client在publish message时需要提供一个”callback“(回调)的queue地址。code如下:
result = channel.queue_declare(exclusive=True) callback_queue = result.method.queue channel.basic_publish(exchange='', routing_key='rpc_queue', properties=pika.BasicProperties( reply_to = callback_queue, ), body=request) # ... and some code to read a response message from the callback_queue ...
2.1 Message properties
AMQP 预定义了14个属性。它们中的绝大多很少会用到。以下几个是平时用的比较多的:
- delivery_mode: 持久化一个Message(通过设定值为2)。其他任意值都是非持久化。请移步RabbitMQ消息队列(三):任务分发机制
- content_type: 描述mime-type 的encoding。比如设置为JSON编码:设置该property为application/json。
- reply_to: 一般用来指明用于回调的queue(Commonly used to name a callback queue)。
- correlation_id: 在请求中关联处理RPC响应(correlate RPC responses with requests)。
3. 相关id Correlation id
在上个小节里,实现方法是对每个RPC请求都会创建一个callback queue。这是不高效的。幸运的是,在这里有一个解决方法:为每个client创建唯一的callback queue。
这又有其他问题了:收到响应后它无法确定是否是它的,因为所有的响应都写到同一个queue了。上一小节的correlation_id在这种情况下就派上用场了:对于每个request,都设置唯一的一个值,在收到响应后,通过这个值就可以判断是否是自己的响应。如果不是自己的响应,就不去处理。
4. 总结
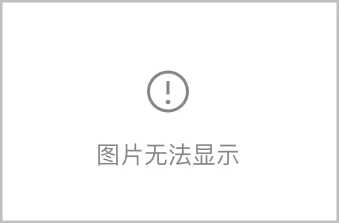
工作流程:
- 当客户端启动时,它创建了匿名的exclusive callback queue.
- 客户端的RPC请求时将同时设置两个properties: reply_to设置为callback queue;correlation_id设置为每个request一个独一无二的值.
- 请求将被发送到an rpc_queue queue.
- RPC端或者说server一直在等待那个queue的请求。当请求到达时,它将通过在reply_to指定的queue回复一个message给client。
- client一直等待callback queue的数据。当message到达时,它将检查correlation_id的值,如果值和它request发送时的一致那么就将返回响应。
5. 最终实现
The code for rpc_server.py:
#!/usr/bin/env python import pika connection = pika.BlockingConnection(pika.ConnectionParameters( host='localhost')) channel = connection.channel() channel.queue_declare(queue='rpc_queue') def fib(n): if n == 0: return 0 elif n == 1: return 1 else: return fib(n-1) + fib(n-2) def on_request(ch, method, props, body): n = int(body) print " [.] fib(%s)" % (n,) response = fib(n) ch.basic_publish(exchange='', routing_key=props.reply_to, properties=pika.BasicProperties(correlation_id = \ props.correlation_id), body=str(response)) ch.basic_ack(delivery_tag = method.delivery_tag) channel.basic_qos(prefetch_count=1) channel.basic_consume(on_request, queue='rpc_queue') print " [x] Awaiting RPC requests" channel.start_consuming()
The server code is rather straightforward:
- (4) As usual we start by establishing the connection and declaring the queue.
- (11) We declare our fibonacci function. It assumes only valid positive integer input. (Don't expect this one to work for big numbers, it's probably the slowest recursive implementation possible).
- (19) We declare a callback for basic_consume, the core of the RPC server. It's executed when the request is received. It does the work and sends the response back.
- (32) We might want to run more than one server process. In order to spread the load equally over multiple servers we need to set theprefetch_count setting.
The code for rpc_client.py:
#!/usr/bin/env python import pika import uuid class FibonacciRpcClient(object): def __init__(self): self.connection = pika.BlockingConnection(pika.ConnectionParameters( host='localhost')) self.channel = self.connection.channel() result = self.channel.queue_declare(exclusive=True) self.callback_queue = result.method.queue self.channel.basic_consume(self.on_response, no_ack=True, queue=self.callback_queue) def on_response(self, ch, method, props, body): if self.corr_id == props.correlation_id: self.response = body def call(self, n): self.response = None self.corr_id = str(uuid.uuid4()) self.channel.basic_publish(exchange='', routing_key='rpc_queue', properties=pika.BasicProperties( reply_to = self.callback_queue, correlation_id = self.corr_id, ), body=str(n)) while self.response is None: self.connection.process_data_events() return int(self.response) fibonacci_rpc = FibonacciRpcClient() print " [x] Requesting fib(30)" response = fibonacci_rpc.call(30) print " [.] Got %r" % (response,)
The client code is slightly more involved:
- (7) We establish a connection, channel and declare an exclusive 'callback' queue for replies.
- (16) We subscribe to the 'callback' queue, so that we can receive RPC responses.
- (18) The 'on_response' callback executed on every response is doing a very simple job, for every response message it checks if thecorrelation_id is the one we're looking for. If so, it saves the response inself.response and breaks the consuming loop.
- (23) Next, we define our main call method - it does the actual RPC request.
- (24) In this method, first we generate a unique correlation_id number and save it - the 'on_response' callback function will use this value to catch the appropriate response.
- (25) Next, we publish the request message, with two properties: reply_to and correlation_id.
- (32) At this point we can sit back and wait until the proper response arrives.
- (33) And finally we return the response back to the user.
开始rpc_server.py:
$ python rpc_server.py [x] Awaiting RPC requests通过client来请求fibonacci数:
$ python rpc_client.py [x] Requesting fib(30)现在这个设计并不是唯一的,但是这个实现有以下优势:
- 如何RPC server太慢,你可以扩展它:启动另外一个RPC server。
- 在client端, 无所进行加锁能同步操作,他所作的就是发送请求等待响应。
我们的code还是挺简单的,并没有尝试去解决更复杂和重要的问题,比如:
- 如果没有server在运行,client需要怎么做?
- RPC应该设置超时机制吗?
- 如果server运行出错并且抛出了异常,需要将这个问题转发到client吗?
- 需要边界检查吗?
尊重原创,转载请注明出处 anzhsoft: http://blog.csdn.net/anzhsoft/article/details/19633107
参考资料:
1. http://www.rabbitmq.com/tutorials/tutorial-six-python.html