Theory Behind Relevance Scoring
Lucene (and thus Elasticsearch) uses the Boolean model to find matching documents, and a formula called the practical scoring function to calculate relevance. This formula borrows concepts from term frequency/inverse document frequency and the vector space model but adds more-modern features like a coordination factor, field length normalization, and term or query clause boosting.
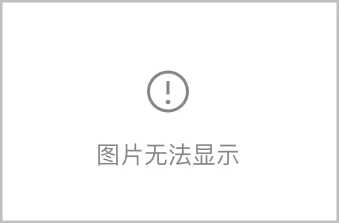
Don’t be alarmed! These concepts are not as complicated as the names make them appear. While this section mentions algorithms, formulae, and mathematical models, it is intended for consumption by mere humans. Understanding the algorithms themselves is not as important as understanding the factors that influence the outcome.
The Boolean model simply applies the AND
, OR
, and NOT
conditions expressed in the query to find all the documents that match. A query for
full AND text AND search AND (elasticsearch OR lucene)
will include only documents that contain all of the terms full
, text
, and search
, and eitherelasticsearch
or lucene
.
This process is simple and fast. It is used to exclude any documents that cannot possibly match the query.
Once we have a list of matching documents, they need to be ranked by relevance. Not all documents will contain all the terms, and some terms are more important than others. The relevance score of the whole document depends (in part) on the weight of each query term that appears in that document.
The weight of a term is determined by three factors, which we already introduced in What Is Relevance?. The formulae are included for interest’s sake, but you are not required to remember them.
How often does the term appear in this document? The more often, the higher the weight. A field containing five mentions of the same term is more likely to be relevant than a field containing just one mention. The term frequency is calculated as follows:
tf(t in d) = √frequency
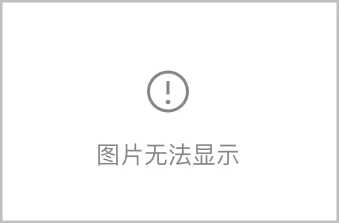
The term frequency ( |
If you don’t care about how often a term appears in a field, and all you care about is that the term is present, then you can disable term frequencies in the field mapping:
How often does the term appear in all documents in the collection? The more often, the lower the weight. Common terms like and
or the
contribute little to relevance, as they appear in most documents, while uncommon terms like elastic
or hippopotamus
help us zoom in on the most interesting documents. The inverse document frequency is calculated as follows:
idf(t) = 1 + log ( numDocs / (docFreq + 1))
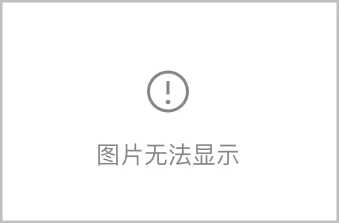