JAX 中文文档(十一)(4)https://developer.aliyun.com/article/1559783
整数和浮点混合提升
当将整数提升为浮点数时,我们可能会从与有符号和无符号整数之间的混合提升相同的思路开始。16 位有符号或无符号整数无法被只有 10 位尾数的 16 位浮点数以全精度表示。因此,将整数提升为比特数加倍的浮点数可能是有道理的:
显示代码单元格源代码 隐藏代码单元格源代码
#@title import networkx as nx import matplotlib.pyplot as plt lattice = { 'i*': ['f*', 'u8', 'i8'], 'f*': ['c*', 'f16'], 'c*': ['c64'], 'u8': ['u16', 'i16', 'f16'], 'u16': ['u32', 'i32', 'f32'], 'u32': ['u64', 'i64', 'f64'], 'i8': ['i16', 'f16'], 'i16': ['i32', 'f32'], 'i32': ['i64', 'f64'], 'f16': ['f32'], 'f32': ['f64', 'c64'], 'f64': ['c128'], 'c64': ['c128'] } graph = nx.from_dict_of_lists(lattice, create_using=nx.DiGraph) pos = { 'i*': [-1.25, 0.5], 'f*': [-0.5, 2], 'c*': [0, 3], 'u8': [0.5, 0], 'u16': [1.5, 0], 'u32': [2.5, 0], 'u64': [3.5, 0], 'i8': [0, 1], 'i16': [1, 1], 'i32': [2, 1], 'i64': [3, 1], 'f16': [0.5, 2], 'f32': [1.5, 2], 'f64': [2.5, 2], 'c64': [2, 3], 'c128': [3, 3], } fig, ax = plt.subplots(figsize=(6, 5)) nx.draw(graph, with_labels=True, node_size=1500, node_color='lightgray', pos=pos, ax=ax) ```</details> 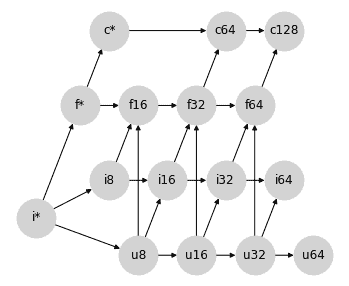 这实际上是 NumPy 类型提升所做的事情,但在这样做时它破坏了图的格性质:例如,对于*{i8, u8}*对,不再有唯一的最小上界:可能性有*i16*和*f16*,这在图上是不可排序的。这事实上是 NumPy 非可结合类型提升的根源。 我们能否提出 NumPy 提升规则的修改,以便满足格性质,并为混合类型提升提供明智的结果?我们在这里可以采取几种方法。 ### 选项 0:将整数/浮点混合精度未定义 为了使行为完全可预测(虽然会损失用户方便性),一个可以辩护的选择是在 Python 标量之外将任何混合整数/浮点数提升保留为未定义状态,停留在前一节的部分格子结构。缺点是用户在操作整数和浮点数数量之间时需要显式类型转换。 ### 选项 1:避免所有精度损失 如果我们的重点是以任何代价避免精度损失,我们可以通过其现有的有符号整数路径将无符号整数提升为浮点数来恢复格子属性: <details class="hide above-input"><summary aria-label="Toggle hidden content">显示代码单元格源代码 隐藏代码单元格源代码</summary> ```py #@title import networkx as nx import matplotlib.pyplot as plt lattice = { 'i*': ['f*', 'u8', 'i8'], 'f*': ['c*', 'f16'], 'c*': ['c64'], 'u8': ['u16', 'i16'], 'u16': ['u32', 'i32'], 'u32': ['u64', 'i64'], 'i8': ['i16', 'f16'], 'i16': ['i32', 'f32'], 'i32': ['i64', 'f64'], 'f16': ['f32'], 'f32': ['f64', 'c64'], 'f64': ['c128'], 'c64': ['c128'] } graph = nx.from_dict_of_lists(lattice, create_using=nx.DiGraph) pos = { 'i*': [-1.25, 0.5], 'f*': [-0.5, 2], 'c*': [0, 3], 'u8': [0.5, 0], 'u16': [1.5, 0], 'u32': [2.5, 0], 'u64': [3.5, 0], 'i8': [0, 1], 'i16': [1, 1], 'i32': [2, 1], 'i64': [3, 1], 'f16': [0.5, 2], 'f32': [1.5, 2], 'f64': [2.5, 2], 'c64': [2, 3], 'c128': [3, 3], } fig, ax = plt.subplots(figsize=(6, 5)) nx.draw(graph, with_labels=True, node_size=1500, node_color='lightgray', pos=pos, ax=ax) ```</details> 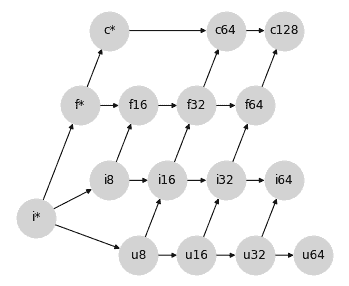 这种方法的一个缺点是它仍然使得`int64`和`uint64`的提升未定义,因为没有标准的浮点类型具有足够的尾数位来表示它们的完整值范围。我们可以放宽精度约束并通过从`i64->f64`和`u64->f64`的连接来完成格子,但这些连接会违反这种提升方案的动机。 第二个缺点是这种格子结构使得很难找到一个合理的位置来插入`bfloat16`(见下文),同时保持格子属性。 对于 JAX 加速器后端来说,这种方法的第三个缺点更为重要,即某些操作会导致比必要宽得多的类型;例如,`uint16` 和 `float16` 之间的混合操作会提升到`float64`,这并不理想。 ### 选项 2:避免大部分比必要更宽的提升 为了解决更广泛类型的不必要提升,我们可以接受整数/浮点数提升可能会导致一些精度损失的可能性,将有符号整数提升为相同宽度的浮点数: <details class="hide above-input"><summary aria-label="Toggle hidden content">显示代码单元格源代码 隐藏代码单元格源代码</summary> ```py #@title import networkx as nx import matplotlib.pyplot as plt lattice = { 'i*': ['f*', 'u8', 'i8'], 'f*': ['c*', 'f16'], 'c*': ['c64'], 'u8': ['u16', 'i16'], 'u16': ['u32', 'i32'], 'u32': ['u64', 'i64'], 'i8': ['i16'], 'i16': ['f16', 'i32'], 'i32': ['f32', 'i64'], 'i64': ['f64'], 'f16': ['f32'], 'f32': ['f64', 'c64'], 'f64': ['c128'], 'c64': ['c128'] } graph = nx.from_dict_of_lists(lattice, create_using=nx.DiGraph) pos = { 'i*': [-1.25, 0.5], 'f*': [-0.5, 2], 'c*': [0, 3], 'u8': [0.5, 0], 'u16': [1.5, 0], 'u32': [2.5, 0], 'u64': [3.5, 0], 'i8': [0, 1], 'i16': [1, 1], 'i32': [2, 1], 'i64': [3, 1], 'f16': [1.5, 2], 'f32': [2.5, 2], 'f64': [3.5, 2], 'c64': [3, 3], 'c128': [4, 3], } fig, ax = plt.subplots(figsize=(6, 5)) nx.draw(graph, with_labels=True, node_size=1500, node_color='lightgray', pos=pos, ax=ax) ```</details> 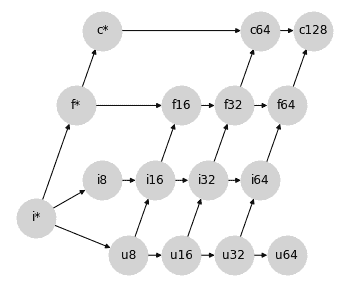 尽管这确实允许在整数和浮点数之间进行精度损失的提升,但这些提升不会误代表结果的*幅度*:虽然浮点数的尾数不足以表示所有值,但指数足以近似它们。 这种方法还允许从`int64`自然提升到`float64`,尽管在此方案中`uint64`仍然无法提升。也就是说,在这里更容易地可以通过其现有的有符号整数路径连接从`u64`到`f64`。 这种提升方案仍然会导致一些比必要更宽的提升路径;例如 `float32` 和 `uint32` 之间的操作将导致 `float64`。此外,这个格子使得很难找到一个合理的地方插入 `bfloat16`(见下文),同时保持格子属性。 ### 选项 3:避免所有比必要更宽的提升 如果我们愿意从根本上改变我们对整数和浮点提升的思维方式,我们可以避免 *所有* 非理想的 64 位提升:就像标量总是遵循数组类型的宽度一样,我们可以使整数总是遵循浮点类型的宽度: <details class="hide above-input"><summary aria-label="Toggle hidden content">显示代码单元源代码 隐藏代码单元源代码</summary> ```py #@title import networkx as nx import matplotlib.pyplot as plt lattice = { 'i*': ['u8', 'i8'], 'f*': ['c*', 'f16'], 'c*': ['c64'], 'u8': ['u16', 'i16'], 'u16': ['u32', 'i32'], 'u32': ['u64', 'i64'], 'i8': ['i16'], 'i16': ['i32'], 'i32': ['i64'], 'i64': ['f*'], 'f16': ['f32'], 'f32': ['f64', 'c64'], 'f64': ['c128'], 'c64': ['c128'] } graph = nx.from_dict_of_lists(lattice, create_using=nx.DiGraph) pos = { 'i*': [-1.25, 0.5], 'f*': [-0.5, 2], 'c*': [0, 3], 'u8': [0.5, 0], 'u16': [1.5, 0], 'u32': [2.5, 0], 'u64': [3.5, 0], 'i8': [0, 1], 'i16': [1, 1], 'i32': [2, 1], 'i64': [3, 1], 'f16': [1.5, 2], 'f32': [2.5, 2], 'f64': [3.5, 2], 'c64': [3, 3], 'c128': [4, 3], } fig, ax = plt.subplots(figsize=(6, 5)) nx.draw(graph, with_labels=True, node_size=1500, node_color='lightgray', pos=pos, ax=ax) ```</details> 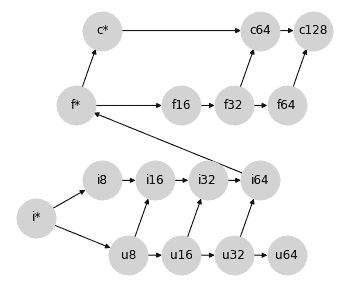 这涉及一种小的手法:之前我们使用 `f*` 表示标量类型。在这个格中,`f*` 可能被应用于混合计算的数组输出。我们不再将 `f*` 视为标量,而是可以将其视为一种具有不同提升规则的特殊类型 `float` 值:在 JAX 中我们称之为 *弱浮点数*;详见下文。 这种方法的优势在于,除了无符号整数外,它避免了 *所有* 比必要更宽的提升:你永远不会得到没有 64 位输入的 f64 输出,也永远不会得到没有 32 位输入的 f32 输出:这对于在加速器上工作时提供了方便的语义,同时避免了无意间生成 64 位值。 这种优先考虑浮点类型的特性类似于 PyTorch 的类型提升行为。这个格子也碰巧生成了一个非常接近 JAX 原始 *临时* 类型提升方案的提升表,该方案不是基于格子的,但具有优先考虑浮点类型的特性。 此外,这个格子还提供了一个自然的位置来插入 `bfloat16`,而无需在 `bf16` 和 `f16` 之间施加排序: <details class="hide above-input"><summary aria-label="Toggle hidden content">显示代码单元源代码 隐藏代码单元源代码</summary> ```py #@title import networkx as nx import matplotlib.pyplot as plt lattice = { 'i*': ['u8', 'i8'], 'f*': ['c*', 'f16', 'bf16'], 'c*': ['c64'], 'u8': ['u16', 'i16'], 'u16': ['u32', 'i32'], 'u32': ['u64', 'i64'], 'i8': ['i16'], 'i16': ['i32'], 'i32': ['i64'], 'i64': ['f*'], 'f16': ['f32'], 'bf16': ['f32'], 'f32': ['f64', 'c64'], 'f64': ['c128'], 'c64': ['c128'] } graph = nx.from_dict_of_lists(lattice, create_using=nx.DiGraph) pos = { 'i*': [-1.25, 0.5], 'f*': [-0.5, 2], 'c*': [0, 3], 'u8': [0.5, 0], 'u16': [1.5, 0], 'u32': [2.5, 0], 'u64': [3.5, 0], 'i8': [0, 1], 'i16': [1, 1], 'i32': [2, 1], 'i64': [3, 1], 'f16': [1.8, 1.7], 'bf16': [1.8, 2.3], 'f32': [3.0, 2], 'f64': [4.0, 2], 'c64': [3.5, 3], 'c128': [4.5, 3], } fig, ax = plt.subplots(figsize=(6, 5)) nx.draw(graph, with_labels=True, node_size=1500, node_color='lightgray', pos=pos, ax=ax) ```</details> 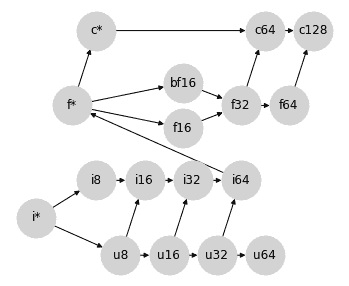 这一点很重要,因为 `f16` 和 `bf16` 不可比较,它们利用其位的方式不同:`bf16` 以较低精度表示更大的范围,而 `f16` 则以较高精度表示更小的范围。 然而,这些优势也伴随着一些权衡: + 混合浮点数/整数提升非常容易产生精度损失:例如,`int64`(最大值为 \(9.2 \times 10^{18}\))可以提升为 `float16`(最大值为 \(6.5 \times 10⁴\)),这意味着大多数可表示的值将变为 `inf`。 + 如上所述,`f*`不再被视为“标量类型”,而是被视为 float64 的不同风味。在 JAX 术语中,这被称为[*弱类型*](https://jax.readthedocs.io/en/latest/type_promotion.html#weakly-typed-values-in-jax),即它表示为 64 位,但在与其他值推广时只弱化到此位宽度。 还请注意,这种方法仍然未解决`uint64`提升问题,尽管将`u64`连接到`f*`可能是合理的。 ## JAX 中的类型提升 在设计 JAX 的类型提升语义时,我们牢记了许多这些想法,并且在几个方面倾向于: 1. 我们选择将 JAX 的类型提升语义约束为满足格属性的图形:这是为了确保结合律和交换律,但也为了允许语义被简洁地描述为 DAG,而不需要一个大表格。 1. 在计算加速器上获益时,我们倾向于避免意外推广到更宽的类型,特别是在涉及浮点值时。 1. 如果需要为了保持(1)和(2),我们可以接受在混合类型提升中潜在的精度损失(但不是幅度损失)。 考虑到这一点,JAX 采用了选项 3。或者更确切地说,选项 3 的一个稍微修改的版本,以建立`u64`与`f*`之间的连接,以创建真正的格。为了清晰起见重新排列节点,JAX 的类型提升格看起来像这样: <details class="hide above-input"><summary aria-label="Toggle hidden content">显示代码单元格源码 隐藏代码单元格源码</summary> ```py #@title import networkx as nx import matplotlib.pyplot as plt lattice = { 'i*': ['u8', 'i8'], 'f*': ['c*', 'f16', 'bf16'], 'c*': ['c64'], 'u8': ['u16', 'i16'], 'u16': ['u32', 'i32'], 'u32': ['u64', 'i64'], 'u64': ['f*'], 'i8': ['i16'], 'i16': ['i32'], 'i32': ['i64'], 'i64': ['f*'], 'f16': ['f32'], 'bf16': ['f32'], 'f32': ['f64', 'c64'], 'f64': ['c128'], 'c64': ['c128'] } graph = nx.from_dict_of_lists(lattice, create_using=nx.DiGraph) pos = { 'i*': [-1.25, 0.5], 'f*': [4.5, 0.5], 'c*': [5, 1.5], 'u8': [0.5, 0], 'u16': [1.5, 0], 'u32': [2.5, 0], 'u64': [3.5, 0], 'i8': [0, 1], 'i16': [1, 1], 'i32': [2, 1], 'i64': [3, 1], 'f16': [5.75, 0.8], 'bf16': [5.75, 0.2], 'f32': [7, 0.5], 'f64': [8, 0.5], 'c64': [7.5, 1.5], 'c128': [8.5, 1.5], } fig, ax = plt.subplots(figsize=(10, 4)) ax.set_ylim(-0.5, 2) nx.draw(graph, with_labels=True, node_size=1500, node_color='lightgray', pos=pos, ax=ax) # ax.patches[12].set_linestyle((0, (2, 4))) ```</details> 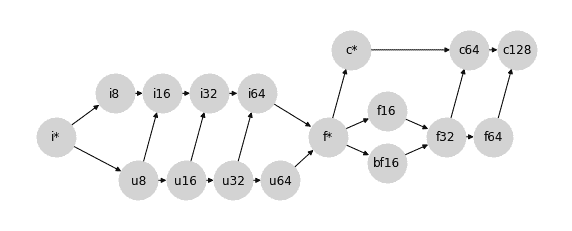 从这种选择产生的行为总结在[JAX 类型提升语义](https://jax.readthedocs.io/en/latest/type_promotion.html)中。特别地,除了包括更大的无符号类型(`u16`、`u32`、`u64`)和一些关于标量/弱类型(`i*`、`f*`、`c*`)行为的细节外,这种类型提升方案与 PyTorch 选择的非常接近。 对于有兴趣的人,附录下面打印了 NumPy、Tensorflow、PyTorch 和 JAX 使用的完整推广表。 ## 附录:示例类型提升表 下面是各种 Python 数组计算库实现的隐式类型提升表的一些示例。 ### NumPy 类型提升 请注意,NumPy 不包括`bfloat16` dtype,并且下表忽略了依赖值影响。 <details class="hide above-input"><summary aria-label="Toggle hidden content">显示代码单元格源码 隐藏代码单元格源码</summary> ```py # @title import numpy as np import pandas as pd from IPython import display np_dtypes = { 'b': np.bool_, 'u8': np.uint8, 'u16': np.uint16, 'u32': np.uint32, 'u64': np.uint64, 'i8': np.int8, 'i16': np.int16, 'i32': np.int32, 'i64': np.int64, 'bf16': 'invalid', 'f16': np.float16, 'f32': np.float32, 'f64': np.float64, 'c64': np.complex64, 'c128': np.complex128, 'i*': int, 'f*': float, 'c*': complex} np_dtype_to_code = {val: key for key, val in np_dtypes.items()} def make_np_zero(dtype): if dtype in {int, float, complex}: return dtype(0) else: return np.zeros(1, dtype=dtype) def np_result_code(dtype1, dtype2): try: out = np.add(make_np_zero(dtype1), make_np_zero(dtype2)) except TypeError: return '-' else: if type(out) in {int, float, complex}: return np_dtype_to_code[type(out)] else: return np_dtype_to_code[out.dtype.type] grid = [[np_result_code(dtype1, dtype2) for dtype2 in np_dtypes.values()] for dtype1 in np_dtypes.values()] table = pd.DataFrame(grid, index=np_dtypes.keys(), columns=np_dtypes.keys()) display.HTML(table.to_html()) ```</details> | | b | u8 | u16 | u32 | u64 | i8 | i16 | i32 | i64 | bf16 | f16 | f32 | f64 | c64 | c128 | i* | f* | c* | | --- | --- | --- | --- | --- | --- | --- | --- | --- | --- | --- | --- | --- | --- | --- | --- | --- | --- | --- | | b | b | u8 | u16 | u32 | u64 | i8 | i16 | i32 | i64 | - | f16 | f32 | f64 | c64 | c128 | i64 | f64 | c128 | | u8 | u8 | u8 | u16 | u32 | u64 | i16 | i16 | i32 | i64 | - | f16 | f32 | f64 | c64 | c128 | u8 | f64 | c128 | | u16 | u16 | u16 | u16 | u32 | u64 | i32 | i32 | i32 | i64 | - | f32 | f32 | f64 | c64 | c128 | u16 | f64 | c128 | | u32 | u32 | u32 | u32 | u32 | u64 | i64 | i64 | i64 | i64 | - | f64 | f64 | f64 | c128 | c128 | u32 | f64 | c128 | | u64 | u64 | u64 | u64 | u64 | u64 | f64 | f64 | f64 | f64 | - | f64 | f64 | f64 | c128 | c128 | u64 | f64 | c128 | | i8 | i8 | i16 | i32 | i64 | f64 | i8 | i16 | i32 | i64 | - | f16 | f32 | f64 | c64 | c128 | i8 | f64 | c128 | | i16 | i16 | i16 | i32 | i64 | f64 | i16 | i16 | i32 | i64 | - | f32 | f32 | f64 | c64 | c128 | i16 | f64 | c128 | | i32 | i32 | i32 | i32 | i64 | f64 | i32 | i32 | i32 | i64 | - | f64 | f64 | f64 | c128 | c128 | i32 | f64 | c128 | | i64 | i64 | i64 | i64 | i64 | f64 | i64 | i64 | i64 | i64 | - | f64 | f64 | f64 | c128 | c128 | i64 | f64 | c128 | | bf16 | - | - | - | - | - | - | - | - | - | - | - | - | - | - | - | - | - | - | | f16 | f16 | f16 | f32 | f64 | f64 | f16 | f32 | f64 | f64 | - | f16 | f32 | f64 | c64 | c128 | f16 | f16 | c64 | | f32 | f32 | f32 | f32 | f64 | f64 | f32 | f32 | f64 | f64 | - | f32 | f32 | f64 | c64 | c128 | f32 | f32 | c64 | | f64 | f64 | f64 | f64 | f64 | f64 | f64 | f64 | f64 | f64 | - | f64 | f64 | f64 | c128 | c128 | f64 | f64 | c128 | | c64 | c64 | c64 | c64 | c128 | c128 | c64 | c64 | c128 | c128 | - | c64 | c64 | c128 | c64 | c128 | c64 | c64 | c64 | | c128 | c128 | c128 | c128 | c128 | c128 | c128 | c128 | c128 | c128 | - | c128 | c128 | c128 | c128 | c128 | c128 | c128 | c128 | | i* | i64 | u8 | u16 | u32 | u64 | i8 | i16 | i32 | i64 | - | f16 | f32 | f64 | c64 | c128 | i64 | f64 | c128 | | f* | f64 | f64 | f64 | f64 | f64 | f64 | f64 | f64 | f64 | - | f16 | f32 | f64 | c64 | c128 | f64 | f64 | c128 | | c* | c128 | c128 | c128 | c128 | c128 | c128 | c128 | c128 | c128 | - | c64 | c64 | c128 | c64 | c128 | c128 | c128 | c128 | ### TensorFlow 类型提升 TensorFlow 避免定义隐式类型提升,除了在有限的情况下,对 Python 标量进行操作。该表格是不对称的,因为在 `tf.add(x, y)` 中,`y` 的类型必须可以强制转换为 `x` 的类型。 <details class="hide above-input"><summary aria-label="Toggle hidden content">显示代码单元格来源 隐藏代码单元格来源</summary> ```py # @title import tensorflow as tf import pandas as pd from IPython import display tf_dtypes = { 'b': tf.bool, 'u8': tf.uint8, 'u16': tf.uint16, 'u32': tf.uint32, 'u64': tf.uint64, 'i8': tf.int8, 'i16': tf.int16, 'i32': tf.int32, 'i64': tf.int64, 'bf16': tf.bfloat16, 'f16': tf.float16, 'f32': tf.float32, 'f64': tf.float64, 'c64': tf.complex64, 'c128': tf.complex128, 'i*': int, 'f*': float, 'c*': complex} tf_dtype_to_code = {val: key for key, val in tf_dtypes.items()} def make_tf_zero(dtype): if dtype in {int, float, complex}: return dtype(0) else: return tf.zeros(1, dtype=dtype) def result_code(dtype1, dtype2): try: out = tf.add(make_tf_zero(dtype1), make_tf_zero(dtype2)) except (TypeError, tf.errors.InvalidArgumentError): return '-' else: if type(out) in {int, float, complex}: return tf_dtype_to_code[type(out)] else: return tf_dtype_to_code[out.dtype] grid = [[result_code(dtype1, dtype2) for dtype2 in tf_dtypes.values()] for dtype1 in tf_dtypes.values()] table = pd.DataFrame(grid, index=tf_dtypes.keys(), columns=tf_dtypes.keys()) display.HTML(table.to_html()) ```</details> | | b | u8 | u16 | u32 | u64 | i8 | i16 | i32 | i64 | bf16 | f16 | f32 | f64 | c64 | c128 | i* | f* | c* | | --- | --- | --- | --- | --- | --- | --- | --- | --- | --- | --- | --- | --- | --- | --- | --- | --- | --- | --- | | b | - | - | - | - | - | - | - | - | - | - | - | - | - | - | - | - | - | - | | u8 | - | u8 | - | - | - | - | - | - | - | - | - | - | - | - | - | u8 | - | - | | u16 | - | - | u16 | - | - | - | - | - | - | - | - | - | - | - | - | u16 | - | - | | u32 | - | - | - | u32 | - | - | - | - | - | - | - | - | - | - | - | u32 | - | - | | u64 | - | - | - | - | u64 | - | - | - | - | - | - | - | - | - | - | u64 | - | - | | i8 | - | - | - | - | - | i8 | - | - | - | - | - | - | - | - | - | i8 | - | - | | i16 | - | - | - | - | - | - | i16 | - | - | - | - | - | - | - | - | i16 | - | - | | i32 | - | - | - | - | - | - | - | i32 | - | - | - | - | - | - | - | i32 | - | - | | i64 | - | - | - | - | - | - | - | - | i64 | - | - | - | - | - | - | i64 | - | - | | bf16 | - | - | - | - | - | - | - | - | - | bf16 | - | - | - | - | - | bf16 | bf16 | - | | f16 | - | - | - | - | - | - | - | - | - | - | f16 | - | - | - | - | f16 | f16 | - | | f32 | - | - | - | - | - | - | - | - | - | - | - | f32 | - | - | - | f32 | f32 | - | | f64 | - | - | - | - | - | - | - | - | - | - | - | - | f64 | - | - | f64 | f64 | - | | c64 | - | - | - | - | - | - | - | - | - | - | - | - | - | c64 | - | c64 | c64 | c64 | | c128 | - | - | - | - | - | - | - | - | - | - | - | - | - | c128 | c128 | c128 | c128 | | i* | - | - | - | - | - | - | - | i32 | - | - | - | - | - | - | - | i32 | - | - | | f* | - | - | - | - | - | - | - | - | - | - | - | f32 | - | - | - | f32 | f32 | - | | c* | - | - | - | - | - | - | - | - | - | - | - | - | - | - | c128 | c128 | c128 | c128 | ### PyTorch 类型提升 注意,torch 不包括大于 `uint8` 的无符号整数类型。除此之外,有关标量/弱类型提升的一些细节,表格接近于 `jax.numpy` 的用法。 <details class="hide above-input"><summary aria-label="Toggle hidden content">显示代码单元源代码 隐藏代码单元源代码</summary> ```py # @title import torch import pandas as pd from IPython import display torch_dtypes = { 'b': torch.bool, 'u8': torch.uint8, 'u16': 'invalid', 'u32': 'invalid', 'u64': 'invalid', 'i8': torch.int8, 'i16': torch.int16, 'i32': torch.int32, 'i64': torch.int64, 'bf16': torch.bfloat16, 'f16': torch.float16, 'f32': torch.float32, 'f64': torch.float64, 'c64': torch.complex64, 'c128': torch.complex128, 'i*': int, 'f*': float, 'c*': complex} torch_dtype_to_code = {val: key for key, val in torch_dtypes.items()} def make_torch_zero(dtype): if dtype in {int, float, complex}: return dtype(0) else: return torch.zeros(1, dtype=dtype) def torch_result_code(dtype1, dtype2): try: out = torch.add(make_torch_zero(dtype1), make_torch_zero(dtype2)) except TypeError: return '-' else: if type(out) in {int, float, complex}: return torch_dtype_to_code[type(out)] else: return torch_dtype_to_code[out.dtype] grid = [[torch_result_code(dtype1, dtype2) for dtype2 in torch_dtypes.values()] for dtype1 in torch_dtypes.values()] table = pd.DataFrame(grid, index=torch_dtypes.keys(), columns=torch_dtypes.keys()) display.HTML(table.to_html()) ```</details> | | b | u8 | u16 | u32 | u64 | i8 | i16 | i32 | i64 | bf16 | f16 | f32 | f64 | c64 | c128 | i* | f* | c* | | --- | --- | --- | --- | --- | --- | --- | --- | --- | --- | --- | --- | --- | --- | --- | --- | --- | --- | --- | | b | b | u8 | - | - | - | i8 | i16 | i32 | i64 | bf16 | f16 | f32 | f64 | c64 | c128 | i64 | f32 | c64 | | u8 | u8 | u8 | - | - | - | i16 | i16 | i32 | i64 | bf16 | f16 | f32 | f64 | c64 | c128 | u8 | f32 | c64 | | u16 | - | - | - | - | - | - | - | - | - | - | - | - | - | - | - | - | - | - | | u32 | - | - | - | - | - | - | - | - | - | - | - | - | - | - | - | - | - | - | | u64 | - | - | - | - | - | - | - | - | - | - | - | - | - | - | - | - | - | - | | i8 | i8 | i16 | - | - | - | i8 | i16 | i32 | i64 | bf16 | f16 | f32 | f64 | c64 | c128 | i8 | f32 | c64 | | i16 | i16 | i16 | - | - | - | i16 | i16 | i32 | i64 | bf16 | f16 | f32 | f64 | c64 | c128 | i16 | f32 | c64 | | i32 | i32 | i32 | - | - | - | i32 | i32 | i32 | i64 | bf16 | f16 | f32 | f64 | c64 | c128 | i32 | f32 | c64 | | i64 | i64 | i64 | - | - | - | i64 | i64 | i64 | i64 | bf16 | f16 | f32 | f64 | c64 | c128 | i64 | f32 | c64 | | bf16 | bf16 | bf16 | - | - | - | bf16 | bf16 | bf16 | bf16 | bf16 | f32 | f32 | f64 | c64 | c128 | bf16 | bf16 | c64 | | f16 | f16 | f16 | - | - | - | f16 | f16 | f16 | f16 | f32 | f16 | f32 | f64 | c64 | c128 | f16 | f16 | c64 | | f32 | f32 | f32 | - | - | - | f32 | f32 | f32 | f32 | f32 | f32 | f32 | f64 | c64 | c128 | f32 | f32 | c64 | | f64 | f64 | f64 | - | - | - | f64 | f64 | f64 | f64 | f64 | f64 | f64 | f64 | c128 | c128 | f64 | f64 | c128 | | c64 | c64 | c64 | - | - | - | c64 | c64 | c64 | c64 | c64 | c64 | c64 | c128 | c64 | c128 | c64 | c64 | c64 | | c128 | c128 | c128 | - | - | - | c128 | c128 | c128 | c128 | c128 | c128 | c128 | c128 | c128 | c128 | c128 | c128 | c128 | | i* | i64 | u8 | - | - | - | i8 | i16 | i32 | i64 | bf16 | f16 | f32 | f64 | c64 | c128 | i64 | f32 | c64 | | f* | f32 | f32 | - | - | - | f32 | f32 | f32 | f32 | bf16 | f16 | f32 | f64 | c64 | c128 | f32 | f64 | c64 | | c* | c64 | c64 | - | - | - | c64 | c64 | c64 | c64 | c64 | c64 | c64 | c128 | c64 | c128 | c64 | c64 | c128 | ### JAX Type Promotion: `jax.numpy` `jax.numpy` follows type promotion rules laid out at https://jax.readthedocs.io/en/latest/type_promotion.html. Here we use `i*`, `f*`, `c*` to indicate both Python scalars and weakly-typed arrays. <details class="hide above-input"><summary aria-label="Toggle hidden content">显示代码单元格源码 隐藏代码单元格源码</summary> ```py # @title from jax import dtypes import jax import jax.numpy as jnp import pandas as pd from IPython import display jax.config.update('jax_enable_x64', True) jnp_dtypes = { 'b': jnp.bool_.dtype, 'u8': jnp.uint8.dtype, 'u16': jnp.uint16.dtype, 'u32': jnp.uint32.dtype, 'u64': jnp.uint64.dtype, 'i8': jnp.int8.dtype, 'i16': jnp.int16.dtype, 'i32': jnp.int32.dtype, 'i64': jnp.int64.dtype, 'bf16': jnp.bfloat16.dtype, 'f16': jnp.float16.dtype, 'f32': jnp.float32.dtype, 'f64': jnp.float64.dtype, 'c64': jnp.complex64.dtype, 'c128': jnp.complex128.dtype, 'i*': int, 'f*': float, 'c*': complex} jnp_dtype_to_code = {val: key for key, val in jnp_dtypes.items()} def make_jnp_zero(dtype): if dtype in {int, float, complex}: return dtype(0) else: return jnp.zeros((), dtype=dtype) def jnp_result_code(dtype1, dtype2): try: out = jnp.add(make_jnp_zero(dtype1), make_jnp_zero(dtype2)) except TypeError: return '-' else: if hasattr(out, 'aval') and out.aval.weak_type: return out.dtype.kind + '*' elif type(out) in {int, float, complex}: return jnp_dtype_to_code[type(out)] else: return jnp_dtype_to_code[out.dtype] grid = [[jnp_result_code(dtype1, dtype2) for dtype2 in jnp_dtypes.values()] for dtype1 in jnp_dtypes.values()] table = pd.DataFrame(grid, index=jnp_dtypes.keys(), columns=jnp_dtypes.keys()) display.HTML(table.to_html()) ```</details> | | b | u8 | u16 | u32 | u64 | i8 | i16 | i32 | i64 | bf16 | f16 | f32 | f64 | c64 | c128 | i* | f* | c* | | --- | --- | --- | --- | --- | --- | --- | --- | --- | --- | --- | --- | --- | --- | --- | --- | --- | --- | --- | | b | b | u8 | u16 | u32 | u64 | i8 | i16 | i32 | i64 | bf16 | f16 | f32 | f64 | c64 | c128 | i* | f* | c* | | u8 | u8 | u8 | u16 | u32 | u64 | i16 | i16 | i32 | i64 | bf16 | f16 | f32 | f64 | c64 | c128 | u8 | f* | c* | | u16 | u16 | u16 | u16 | u32 | u64 | i32 | i32 | i32 | i64 | bf16 | f16 | f32 | f64 | c64 | c128 | u16 | f* | c* | | u32 | u32 | u32 | u32 | u32 | u64 | i64 | i64 | i64 | i64 | bf16 | f16 | f32 | f64 | c64 | c128 | u32 | f* | c* | | u64 | u64 | u64 | u64 | u64 | u64 | f* | f* | f* | f* | bf16 | f16 | f32 | f64 | c64 | c128 | u64 | f* | c* | | i8 | i8 | i16 | i32 | i64 | f* | i8 | i16 | i32 | i64 | bf16 | f16 | f32 | f64 | c64 | c128 | i8 | f* | c* | | i16 | i16 | i16 | i32 | i64 | f* | i16 | i16 | i32 | i64 | bf16 | f16 | f32 | f64 | c64 | c128 | i16 | f* | c* | | i32 | i32 | i32 | i32 | i64 | f* | i32 | i32 | i32 | i64 | bf16 | f16 | f32 | f64 | c64 | c128 | i32 | f* | c* | | i64 | i64 | i64 | i64 | i64 | f* | i64 | i64 | i64 | i64 | bf16 | f16 | f32 | f64 | c64 | c128 | i64 | f* | c* | | bf16 | bf16 | bf16 | bf16 | bf16 | bf16 | bf16 | bf16 | bf16 | bf16 | bf16 | f32 | f32 | f64 | c64 | c128 | bf16 | bf16 | c64 | | f16 | f16 | f16 | f16 | f16 | f16 | f16 | f16 | f16 | f16 | f32 | f16 | f32 | f64 | c64 | c128 | f16 | f16 | c64 | | f32 | f32 | f32 | f32 | f32 | f32 | f32 | f32 | f32 | f32 | f32 | f32 | f32 | f64 | c64 | c128 | f32 | f32 | c64 | | f64 | f64 | f64 | f64 | f64 | f64 | f64 | f64 | f64 | f64 | f64 | f64 | f64 | f64 | c128 | c128 | f64 | f64 | c128 | | c64 | c64 | c64 | c64 | c64 | c64 | c64 | c64 | c64 | c64 | c64 | c64 | c64 | c128 | c64 | c128 | c64 | c64 | c64 | | c128 | c128 | c128 | c128 | c128 | c128 | c128 | c128 | c128 | c128 | c128 | c128 | c128 | c128 | c128 | c128 | c128 | c128 | c128 | | i* | i* | u8 | u16 | u32 | u64 | i8 | i16 | i32 | i64 | bf16 | f16 | f32 | f64 | c64 | c128 | i* | f* | c* | | f* | f* | f* | f* | f* | f* | f* | f* | f* | f* | bf16 | f16 | f32 | f64 | c64 | c128 | f* | f* | c* | | c* | c* | c* | c* | c* | c* | c* | c* | c* | c* | c64 | c64 | c64 | c128 | c64 | c128 | c* | c* | c* | ### JAX 类型提升:`jax.lax` `jax.lax` 是较低级的库,不执行任何隐式类型提升。在这里,我们使用 `i*`、`f*`、`c*` 来表示 Python 标量和弱类型数组。 <details class="hide above-input"><summary aria-label="Toggle hidden content">显示代码单元格源代码 隐藏代码单元格源代码</summary> ```py # @title from jax import dtypes import jax import jax.numpy as jnp import pandas as pd from IPython import display jax.config.update('jax_enable_x64', True) jnp_dtypes = { 'b': jnp.bool_.dtype, 'u8': jnp.uint8.dtype, 'u16': jnp.uint16.dtype, 'u32': jnp.uint32.dtype, 'u64': jnp.uint64.dtype, 'i8': jnp.int8.dtype, 'i16': jnp.int16.dtype, 'i32': jnp.int32.dtype, 'i64': jnp.int64.dtype, 'bf16': jnp.bfloat16.dtype, 'f16': jnp.float16.dtype, 'f32': jnp.float32.dtype, 'f64': jnp.float64.dtype, 'c64': jnp.complex64.dtype, 'c128': jnp.complex128.dtype, 'i*': int, 'f*': float, 'c*': complex} jnp_dtype_to_code = {val: key for key, val in jnp_dtypes.items()} def make_jnp_zero(dtype): if dtype in {int, float, complex}: return dtype(0) else: return jnp.zeros((), dtype=dtype) def jnp_result_code(dtype1, dtype2): try: out = jax.lax.add(make_jnp_zero(dtype1), make_jnp_zero(dtype2)) except TypeError: return '-' else: if hasattr(out, 'aval') and out.aval.weak_type: return out.dtype.kind + '*' elif type(out) in {int, float, complex}: return jnp_dtype_to_code[type(out)] else: return jnp_dtype_to_code[out.dtype] grid = [[jnp_result_code(dtype1, dtype2) for dtype2 in jnp_dtypes.values()] for dtype1 in jnp_dtypes.values()] table = pd.DataFrame(grid, index=jnp_dtypes.keys(), columns=jnp_dtypes.keys()) display.HTML(table.to_html()) ```</details> | | b | u8 | u16 | u32 | u64 | i8 | i16 | i32 | i64 | bf16 | f16 | f32 | f64 | c64 | c128 | i* | f* | c* | | --- | --- | --- | --- | --- | --- | --- | --- | --- | --- | --- | --- | --- | --- | --- | --- | --- | --- | --- | | b | - | - | - | - | - | - | - | - | - | - | - | - | - | - | - | - | - | - | | u8 | - | u8 | - | - | - | - | - | - | - | - | - | - | - | - | - | - | - | - | | u16 | - | - | u16 | - | - | - | - | - | - | - | - | - | - | - | - | - | - | - | | u32 | - | - | - | u32 | - | - | - | - | - | - | - | - | - | - | - | - | - | - | | u64 | - | - | - | - | u64 | - | - | - | - | - | - | - | - | - | - | - | - | - | | i8 | - | - | - | - | - | i8 | - | - | - | - | - | - | - | - | - | - | - | - | | i16 | - | - | - | - | - | - | i16 | - | - | - | - | - | - | - | - | - | - | - | | i32 | - | - | - | - | - | - | - | i32 | - | - | - | - | - | - | - | - | - | - | | i64 | - | - | - | - | - | - | - | - | i64 | - | - | - | - | - | - | i64 | - | - | | bf16 | - | - | - | - | - | - | - | - | - | bf16 | - | - | - | - | - | - | - | - | | f16 | - | - | - | - | - | - | - | - | - | - | f16 | - | - | - | - | - | - | - | | f32 | - | - | - | - | - | - | - | - | - | - | - | f32 | - | - | - | - | - | - | | f64 | - | - | - | - | - | - | - | - | - | - | - | - | f64 | - | - | - | f64 | - | | c64 | - | - | - | - | - | - | - | - | - | - | - | - | - | c64 | - | - | - | - | | c128 | - | - | - | - | - | - | - | - | - | - | - | - | - | - | c128 | - | - | c128 | | i* | - | - | - | - | - | - | - | - | i64 | - | - | - | - | - | - | i* | - | - | | f* | - | - | - | - | - | - | - | - | - | - | - | - | f64 | - | - | - | f* | - | | c* | - | - | - | - | - | - | - | - | - | - | - | - | - | - | c128 | - | - | c* | return '-' else: if hasattr(out, 'aval') and out.aval.weak_type: return out.dtype.kind + '*' elif type(out) in {int, float, complex}: return jnp_dtype_to_code[type(out)] else: return jnp_dtype_to_code[out.dtype] grid = [[jnp_result_code(dtype1, dtype2) for dtype2 in jnp_dtypes.values()] for dtype1 in jnp_dtypes.values()] table = pd.DataFrame(grid, index=jnp_dtypes.keys(), columns=jnp_dtypes.keys()) display.HTML(table.to_html()) ```</details> | | b | u8 | u16 | u32 | u64 | i8 | i16 | i32 | i64 | bf16 | f16 | f32 | f64 | c64 | c128 | i* | f* | c* | | --- | --- | --- | --- | --- | --- | --- | --- | --- | --- | --- | --- | --- | --- | --- | --- | --- | --- | --- | | b | - | - | - | - | - | - | - | - | - | - | - | - | - | - | - | - | - | - | | u8 | - | u8 | - | - | - | - | - | - | - | - | - | - | - | - | - | - | - | - | | u16 | - | - | u16 | - | - | - | - | - | - | - | - | - | - | - | - | - | - | - | | u32 | - | - | - | u32 | - | - | - | - | - | - | - | - | - | - | - | - | - | - | | u64 | - | - | - | - | u64 | - | - | - | - | - | - | - | - | - | - | - | - | - | | i8 | - | - | - | - | - | i8 | - | - | - | - | - | - | - | - | - | - | - | - | | i16 | - | - | - | - | - | - | i16 | - | - | - | - | - | - | - | - | - | - | - | | i32 | - | - | - | - | - | - | - | i32 | - | - | - | - | - | - | - | - | - | - | | i64 | - | - | - | - | - | - | - | - | i64 | - | - | - | - | - | - | i64 | - | - | | bf16 | - | - | - | - | - | - | - | - | - | bf16 | - | - | - | - | - | - | - | - | | f16 | - | - | - | - | - | - | - | - | - | - | f16 | - | - | - | - | - | - | - | | f32 | - | - | - | - | - | - | - | - | - | - | - | f32 | - | - | - | - | - | - | | f64 | - | - | - | - | - | - | - | - | - | - | - | - | f64 | - | - | - | f64 | - | | c64 | - | - | - | - | - | - | - | - | - | - | - | - | - | c64 | - | - | - | - | | c128 | - | - | - | - | - | - | - | - | - | - | - | - | - | - | c128 | - | - | c128 | | i* | - | - | - | - | - | - | - | - | i64 | - | - | - | - | - | - | i* | - | - | | f* | - | - | - | - | - | - | - | - | - | - | - | - | f64 | - | - | - | f* | - | | c* | - | - | - | - | - | - | - | - | - | - | - | - | - | - | c128 | - | - | c* |