ModelScope我的推理速度,device为CPU和GPU是完全一样的,有点奇怪。我发现tensorflow 1.15需要cuda10 是不是我的cuda11.4不兼容呢?print(pipeline_ins(input=inputs,batch_size=100,device='cpu')), GPU版本
conda create -n py37testmaas python=3.7
pip install cryptography==3.4.8 tensorflow-gpu==1.15.5 torch==1.11.0 torchvision==0.12.0 torchaudio==0.11.0
pip install "modelscope[nlp]" -f https://modelscope.oss-cn-beijing.aliyuncs.com/releases/repo.html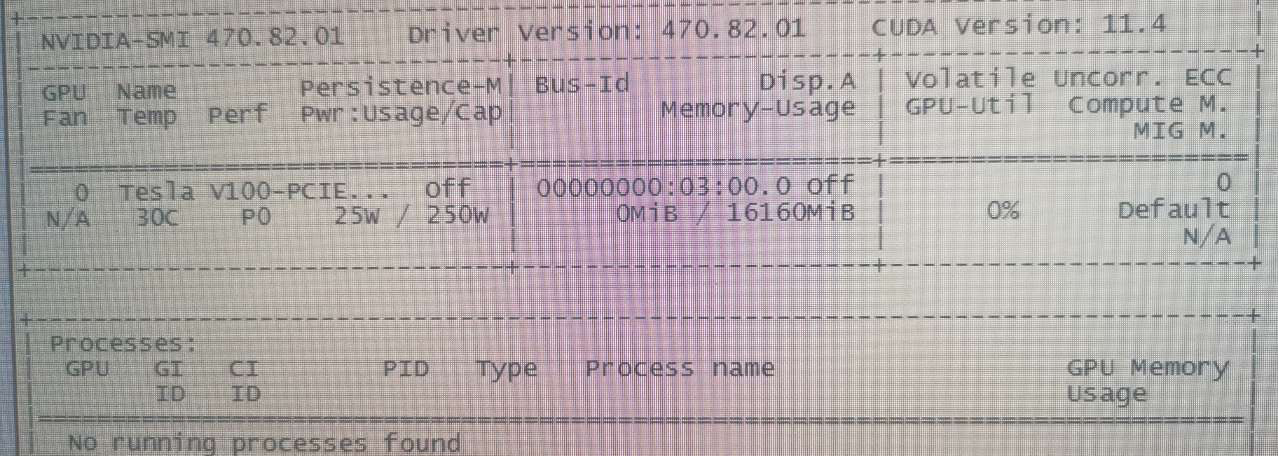
直接使用官方的模型from modelscope.pipelines import pipeline
from modelscope.utils.constant import Tasks
task = Tasks.token_classification
model = 'damo/mgeo_geographic_elements_tagging_chinese_base'
inputs = '浙江省杭州市余杭区阿里巴巴西溪园区'
pipeline_ins = pipeline(
task=task, model=model)
print(pipeline_ins(input=inputs))
首先感谢您提供了详细的描述和图片,看起来确实有一些异常的表现。
关于推理速度的问题,按照一般的预期来说,应该是在GPU环境下推理的速度会更快一些,但是从您提供的结果来看似乎CPU和GPU之间的表现差距不大甚至完全一致。这可能是由于多个原因造成的:
建议您可以通过以下方式进行排查:
nvidia-smi
即可显示当前机器上的所有GPU设备及其状态;tf.test.is_gpu_available()
就可以得知当前TensorFlow是否成功检测到了GPU设备;我看到了您的截图,看起来像是遇到了一些问题。
关于 Tensorflow 1.15 和 cuda 11.4 不兼容的问题,首先需要指出的是,Tensorflow 1.15 只支持 cuda 10.x 的驱动程序。您可以尝试降低 cuda 版本至 cuda 10.0 以上,以确保与 Tensorflow 1.15 兼容。
此外,建议在安装过程中不要直接使用 conda 创建虚拟环境,因为有些依赖可能无法被正确安装。您可以尝试使用 pip 命令来创建一个新的 Python 环境,并安装 TensorFlow 和 PyTorch:
pip install numpy
pip install tensorflow==1.15.5
pip install torch==1.11.0+cu111 torchvision==0.12.0+cu111 torchaudio==0.11.0 -f https://download.pytorch.org/whl/torch_stable.html
pip install transformers
pip install modelscope[nlp] -f https://modelscope.oss-cn-beijing.aliyuncs.com/releases/repo.html
从你提供的信息来看,你的问题可能与TensorFlow的版本和CUDA的版本不兼容有关。TensorFlow 1.15版本需要CUDA 10,而你的CUDA版本是11.4,这可能是导致问题的原因。
你可以尝试以下几种解决方法:
降级你的CUDA版本:将CUDA版本降至10,以匹配TensorFlow 1.15的版本。
升级你的TensorFlow版本:将TensorFlow版本升至最新版本,以兼容CUDA 11.4。
使用支持CUDA 11.4的TensorFlow版本:例如,TensorFlow 2.x版本支持CUDA 11.4。
我这边cpu和gpu的推理时间也是相差不大,但gpu是用了的。有时候模型比较小的话,会存在这种情况。,此回答整理自钉群“魔搭ModelScope开发者联盟群 ①”