转载自:AI前线
一. Flume 简介
Apache Flume 是 Cloudera 公司开源的一款分布式、可靠、可用的服务,可用于从多种不同数据源收集、聚集、移动大量日志数据到集中数据存储中;它通过事务机制提供了可靠的消息传输支持,并自带负载均衡机制来支撑水平扩展。尤其近几年随着 Flume 的不断被完善以及升级版本的逐一推出,特别是 flume-ng 的推出,以及 Flume 内部的各种组件不断丰富,用户在开发的过程中使用的便利性得到很大的改善,现已成为 Apache 顶级社区项目之一。
二. 中国民生银行 Flume 实践
中国民生银行服务器的操作系统种类众多,除 Linux 外,部分生产系统仍采用 AIX 和 HP-UNIX 操作系统,由于在 AIX 和 HP-UNIX 无法使用 Logstash 作为日志采集端,在大数据基础平台产品团队经过一系列选型后,采用 Flume 作为 AIX 和 HP-UNIX 操作系统上日志采集端。
2016 年我们在测试环境进行试验,使用的版本是 Apache Flume 1.6,在使用 Taildir Source 组件和核心组件的过程中,发现其无法完全满足我们的需求,例如:
- 若 filegroup 路径中包含正则表达式,则无法获取文件的完整路径,在日志入到 Elasticsearch 后无法定位日志的路径;
- Taildir Source 不支持将多行合并为一个 event,只能一行一行读取文件;
- filegroup 配置中不支持目录包含正则表达式,不便配置包含多个日期并且日期自动增长的目录,例如 /app/logs/yyyymmdd/appLog.log;
- 在使用 Host Interceptor 时,发现只能保留主机名或者是 IP,二者无法同时保留。
在研究 Flume 源码之后,我们在源码上扩展开发。截至目前,我们为开源社区贡献了 4 个 Patch,其中 FLUME-2955 已被社区 Merge 并在 1.7 版本中发布,另外我们在 Github 上开放了一个版本,将 FLUME-2960/2961/3187 三个 Patch 合并到 Flume 1.7 上,欢迎大家下载使用,
Github 地址:
https://github.com/tinawenqiao/flume,分支名 trunk-cmbc。
接下来本文将对每个 Issue 进行详细介绍:
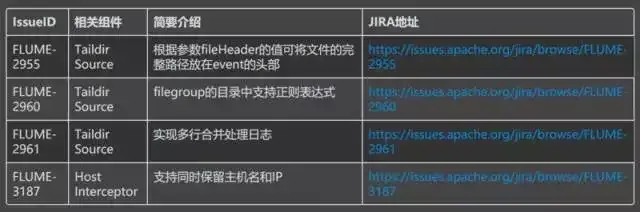
三. FLUME-2955
3.1 问题和需求
为了采集后缀为 log 的日志文件,filegroups 设置如下:
agent.sources.s1.type = org.apache.flume.source.taildir.TaildirSource
agent.sources.s1.filegroups = f1
agent.sources.s1.filegroups.f1 = /app/logs/.*.log
注:安卓手机端读者查看代码时可左右滑动阅读完整代码
若 /app/logs 目录中存在 a.log、b.log、c.log 三个文件,在 Flume 1.6 版本中,虽然可以通过 headers.\.\在 event 的 header 里放入自定义的 key 和 value,但是由于正则表达式匹配上了目录中多个文件,所以无法通过该方法设置,这样导致日志数据入到 Elasticsearch 后,用户从 Kibana 从查询时无法定位到数据所在的日志文件路径。
3.2 解决办法
增加 fileHeader 和 fileHeaderKey 两个参数,两个参数含义分别是:

修改类 ReliableTaildirEventReader 中 readEvents() 方法,根据配置文件的值,选择是否在 event 的 header 里加入文件的路径,主要代码如下:
Map<String, String> headers = currentFile.getHeaders();
if (annotateFileName || (headers != null && !headers.isEmpty())) {
for (Event event : events) {
if (headers != null && !headers.isEmpty()) {
event.getHeaders().putAll(headers);
}
if (annotateFileName) {
event.getHeaders().put(fileNameHeader, currentFile.getPath());
}
}
}
3.3 相关配置示例
agent.sources.s1.type = org.apache.flume.source.taildir.TaildirSource
agent.sources.s1.filegroups = f1
agent.sources.s1.filegroups.f1 = /app/logs/.*.log
agent.sources.s1.fileHeader = true
agent.sources.s1.fileHeaderKey = path
四. FLUME-2960
4.1 问题和需求
在实际应用写日志时,很多系统是根据日期生成日期目录,每个日期目录中包含一个或多个日志文件,因此存在:
/app/logs/20170101/、/app/logs/20170102/、/app/logs/20170103/
等多个目录,且 /app/logs/ 目录下每天会自动生成新的日期目录,但是根据 Taildir Source 中 filegroups.\的描述,只支持文件名带正则,因此 1.6 版本的 Taildir Source 无法满足该需求。
4.2 解决办法
增加 filegroups.\.parentDir 和 filegroups.\.filePattern 两个参数,两个参数含义分别是:

修改类 TaildirMatcher 中匹配文件的方法,相关代码如下:
private List<File> getMatchingFilesNoCache() {
final List<File> result = Lists.newArrayList();
try {
Set options = EnumSet.of(FOLLOW_LINKS);
Files.walkFileTree(Paths.get(parentDir.toString()), options, Integer.MAX_VALUE,
new SimpleFileVisitor<Path>() {
@Override
public FileVisitResult visitFile(Path file, BasicFileAttributes attrs) {
if (fileMatcher.matches(file.toAbsolutePath())) {
result.add(file.toFile());
}
return FileVisitResult.CONTINUE;
}
@Override
public FileVisitResult visitFileFailed(Path file, IOException exc) {
return FileVisitResult.CONTINUE;
}
});
}
...
}
另外进行了配置参数的兼容性处理,用户仍可保留以前的 filegroups 配置,不需单独配置 parentDir 和 filePattern,程序会将 filegroups 中的文件的目录赋值给 parentDir,文件名赋值给 filePattern。
需要注意的是:在 Taildir Source 中有个参数 cachePatternMatching,默认值是 true,其作用是缓存正则匹配的文件列表和消费文件的顺序,若目录中文件较多时,使用正则匹配比较耗时,设置该参数可提高性能,当发现文件的目录修改后会刷新缓存列表。由于 filePattern 中可包含目录,若 cachePatternMatching 设为 true,在 filePattern 的子目录中新增文件,parentDir 的修改时间不变,此时新增的日志文件不能被跟踪到,因此,建议在 filePattern 包含目录的情况下,将 cachePatternMatching 设置为 false。
4.3 相关配置示例
agent.sources.s2.type = org.apache.flume.source.taildir.TaildirSource
agent.sources.s2.filegroups = f1 f2
agent.sources.s2.filegroups.f1.parentDir = /app/log/
agent.sources.s2.filegroups.f1.filePattern = /APP.log.\\d{8}
agent.sources.s2.filegroups.f2.parentDir = /app/log/
agent.sources.s2.filegroups.f2.filePattern = /\\w/.*log
agent.sources.s2.cachePatternMatching = false
五. FLUME-2961
5.1 问题和需求
Taildir Source 按行读取日志,把每一行作为内容放入 flume event 的 body 中,对于以下这种每行就可以结束的日志处理没有问题:
13 七月 2016 23:37:30,580 INFO [lifecycleSupervisor-1-0] (org.apache.flume.node.PollingPropertiesFileConfigurationProvider.start:62) - Configuration provider starting
13 七月 2016 23:37:30,585 INFO [conf-file-poller-0] (org.apache.flume.node.PollingPropertiesFileConfigurationProvider$FileWatcherRunnable.run:134) - Reloading configuration file:conf/taildir.conf
13 七月 2016 23:37:30,592 INFO [conf-file-poller-0] (org.apache.flume.conf.FlumeConfiguration$AgentConfiguration.addProperty:1013) - Processing:s1
但是对于类似 Java Stacktrace 的日志,如果按上述处理,以下日志被截断成 9 个 flume event(一共 9 行)输出,而我们希望这样的日志记录,要作为 1 个 flume event,而不是 9 个输出:
13 七月 2016 23:37:41,942 ERROR [SinkRunner-PollingRunner-DefaultSinkProcessor] (org.apache.flume.sink.kafka.KafkaSink.process:229) - Failed to publish events
java.util.concurrent.ExecutionException: org.apache.kafka.common.errors.RecordTooLargeException: The message is 2000067 bytes when serialized which is larger than the maximum request size you have configured with the max.request.size configuration.
at org.apache.kafka.clients.producer.KafkaProducer$FutureFailure.<init>(KafkaProducer.java:686)
at org.apache.kafka.clients.producer.KafkaProducer.send(KafkaProducer.java:449)
at org.apache.flume.sink.kafka.KafkaSink.process(KafkaSink.java:200)
at org.apache.flume.sink.DefaultSinkProcessor.process(DefaultSinkProcessor.java:67)
at org.apache.flume.SinkRunner$PollingRunner.run(SinkRunner.java:145)
at java.lang.Thread.run(Thread.java:745)
Caused by: org.apache.kafka.common.errors.RecordTooLargeException: The message is 2000067 bytes when serialized which is larger than the maximum request size you have configured with the max.request.size configuration.
5.2 解决办法
设计一个 buffer event 缓存多行内容,仿照 Logstash 的 codec/mulitline 插件配置,增加了如下参数:
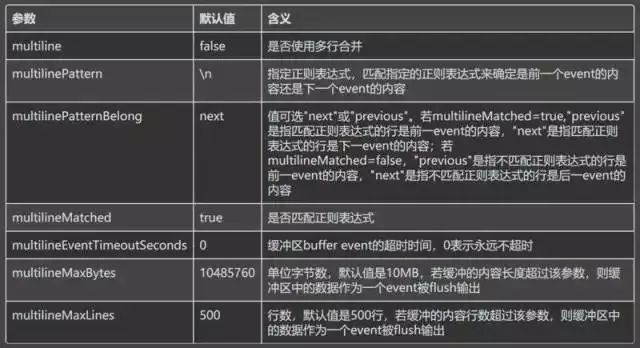
主要修改了类 TailFile 里的 readEvents() 方法,相关代码如下:
if (this.multiline) {
if (raf != null) { // when file has not closed yet
boolean match = this.multilinePatternMatched;
while (events.size() < numEvents) {
LineResult line = readLine();
if (line == null) {
break;
}
Event event = null;
logger.debug("TailFile.readEvents: Current line = " + new String(line.line) +
". Current time : " + new Timestamp(System.currentTimeMillis()) +
". Pos:" + pos +
". LineReadPos:" + lineReadPos + ",raf.getPointer:" + raf.getFilePointer());
switch (this.multilinePatternBelong) {
case "next":
event = readMultilineEventNext(line, match);
break;
case "previous":
event = readMultilineEventPre(line, match);
break;
default:
break;
}
if (event != null) {
events.add(event);
}
if (bufferEvent != null) {
if (bufferEvent.getBody().length >= multilineMaxBytes
|| Integer.parseInt(bufferEvent.getHeaders().get("lineCount")) == multilineMaxLines) {
flushBufferEvent(events);
}
}
}
}
if (needFlushTimeoutEvent()) {
flushBufferEvent(events);
}
}
合并多行处理的方法代码如下:
private Event readMultilineEventPre(LineResult line, boolean match)
throws IOException {
Event event = null;
Matcher m = multilinePattern.matcher(new String(line.line));
boolean find = m.find();
match = (find && match) || (!find && !match);
byte[] lineBytes = toOriginBytes(line);
if (match) {
/** If matched, merge it to the buffer event. */
mergeEvent(line);
} else {
/**
* If not matched, this line is not part of previous event when the buffer event is not null.
* Then create a new event with buffer event's message and put the current line into the
* cleared buffer event.
*/
if (bufferEvent != null) {
event = EventBuilder.withBody(bufferEvent.getBody());
}
bufferEvent = null;
bufferEvent = EventBuilder.withBody(lineBytes);
if (line.lineSepInclude) {
bufferEvent.getHeaders().put("lineCount", "1");
} else {
bufferEvent.getHeaders().put("lineCount", "0");
}
long now = System.currentTimeMillis();
bufferEvent.getHeaders().put("time", Long.toString(now));
}
return event;
}
private Event readMultilineEventNext(LineResult line, boolean match)
throws IOException {
Event event = null;
Matcher m = multilinePattern.matcher(new String(line.line));
boolean find = m.find();
match = (find && match) || (!find && !match);
if (match) {
/** If matched, merge it to the buffer event. */
mergeEvent(line);
} else {
/**
* If not matched, this line is not part of next event. Then merge the current line into the
* buffer event and create a new event with the merged message.
*/
mergeEvent(line);
event = EventBuilder.withBody(bufferEvent.getBody());
bufferEvent = null;
}
return event;
}
3.3 相关配置示例
agent.sources.s3.multiline = true
agent.sources.s3.multilinePattern = ^AGENT_IP:
agent.sources.s3.multilinePatternBelong = previous
agent.sources.s3.multilineMatched = false
agent.sources.s3.multilineEventTimeoutSeconds = 120
agent.sources.s3.multilineMaxBytes = 3145728
agent.sources.s3.multilineMaxLines = 3000
六. FLUME-3187
6.1 问题和需求
为了获取 Flume agent 所在机器的主机名或 IP,我们使用了主机名拦截器 (Host Interceptor),但是根据主机名拦截器的定义,只能保留主机名和 IP 中的一种,无法同时保留主机名和 IP。
Host Interceptor
This interceptor inserts the hostname or IP address of the host that this agent is running on. It inserts a header with key host or a configured key whose value is the hostname or IP address of the host, based on configuration.
6.2 解决办法
将原来的 useIP 参数扩展,增加一个参数 useHostname,若同时设置为 true,可同时保留主机名和 IP;另外支持自定义主机名和 IP 地址在 event header 里的 key,参数如下:
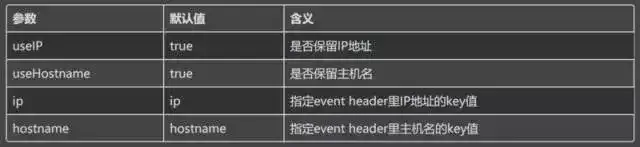
修改了类 HostInterceptor 中的构造方法和拦截方法,相关代码如下:
addr = InetAddress.getLocalHost();
if (useIP) {
ip = addr.getHostAddress();
}
if (useHostname) {
hostname = addr.getCanonicalHostName();
}
6.3 相关配置示例
agent.sources.s4.interceptors = i1
agent.sources.s4.interceptors.i1.type = host
agent.sources.s4.interceptors.i1.useIP = true
agent.sources.s4.interceptors.i1.useHostname = true
agent.sources.s4.interceptors.i1.ip = ip
agent.sources.s4.interceptors.i1.hostname = hostname
总结
目前上述 4 个 Patch 在我行 A 类和 B 类生产系统已实际运行使用,“拥抱开源,回馈开源”,我们用的是开源软件,我们希望也能对开源软件做出贡献。后续我们将分享我行 ELK 日志平台架构演进的详细细节,敬请大家关注!