---------------------------------------------------------------------------------------------------------------
[版权申明:本文系作者原创,转载请注明出处]
文章出处:http://blog.csdn.net/sdksdk0/article/details/51628874
作者:朱培
---------------------------------------------------------------------------------------------------------------
本文是结合hadoop中的mapreduce来对用户数据进行分析,统计用户的手机号码、上行流量、下行流量、总流量的信息,同时可以按照总流量大小对用户进行分组排序等。是一个非常简洁易用的hadoop项目,主要用户进一步加强对MapReduce的理解及实际应用。文末提供源数据采集文件和系统源码。
本案例非常适合hadoop初级人员学习以及想入门大数据、云计算、数据分析等领域的朋友进行学习。
一、待分析的数据源
以下是一个待分析的文本文件,里面有非常多的用户浏览信息,保扩用户手机号码,上网时间,机器序列号,访问的IP,访问的网站,上行流量,下行流量,总流量等信息。这里只截取一小段,具体文件在文末提供下载链接。
二、基本功能实现
private long upFlow; private long dFlow; private long sumFlow;然后就是各种右键生成get,set方法,还要toString(),以及生成构造函数,(千万记得要生成一个空的构造函数,不然后面进行分析的时候会报错)。
package cn.tf.flow; import java.io.DataInput; import java.io.DataOutput; import java.io.IOException; import org.apache.hadoop.io.Writable; import org.apache.hadoop.io.WritableComparable; public class FlowBean implements WritableComparable<FlowBean>{ private long upFlow; private long dFlow; private long sumFlow; public long getUpFlow() { return upFlow; } public void setUpFlow(long upFlow) { this.upFlow = upFlow; } public long getdFlow() { return dFlow; } public void setdFlow(long dFlow) { this.dFlow = dFlow; } public long getSumFlow() { return sumFlow; } public void setSumFlow(long sumFlow) { this.sumFlow = sumFlow; } public FlowBean(long upFlow, long dFlow) { super(); this.upFlow = upFlow; this.dFlow = dFlow; this.sumFlow = upFlow+dFlow; } @Override public void readFields(DataInput in) throws IOException { upFlow=in.readLong(); dFlow=in.readLong(); sumFlow=in.readLong(); } @Override public void write(DataOutput out) throws IOException { out.writeLong(upFlow); out.writeLong(dFlow); out.writeLong(sumFlow); } public FlowBean() { super(); } @Override public String toString() { return upFlow + "\t" + dFlow + "\t" + sumFlow; } @Override public int compareTo(FlowBean o) { return this.sumFlow>o.getSumFlow() ? -1:1; } }
然后就是这个统计的代码了,新建一个FlowCount.java.在这个类里面,我直接把Mapper和Reduce写在同一个类里面了,如果按规范的要求应该是要分开写的。
public static class FlowCountMapper extends Mapper<LongWritable, Text, Text, FlowBean> { @Override protected void map(LongWritable key, Text value, Context context) throws IOException, InterruptedException { // 拿到这行的内容转成string String line = value.toString(); String[] fields = StringUtils.split(line, "\t"); try { if (fields.length > 3) { // 获得手机号及上下行流量字段值 String phone = fields[1]; long upFlow = Long.parseLong(fields[fields.length - 3]); long dFlow = Long.parseLong(fields[fields.length - 2]); // 输出这一行的处理结果,key为手机号,value为流量信息bean context.write(new Text(phone), new FlowBean(upFlow, dFlow)); } else { return; } } catch (Exception e) { } } }
在reduce中队数据进行整理,统计
public static class FlowCountReducer extends Reducer<Text, FlowBean, Text, FlowBean> { @Override protected void reduce(Text key, Iterable<FlowBean> values, Context context) throws IOException, InterruptedException { long upSum = 0; long dSum = 0; for (FlowBean bean : values) { upSum += bean.getUpFlow(); dSum += bean.getdFlow(); } FlowBean resultBean = new FlowBean(upSum, dSum); context.write(key, resultBean); } }
public static void main(String[] args) throws Exception { Configuration conf = new Configuration(); Job job = Job.getInstance(conf); job.setJarByClass(FlowCount.class); job.setMapperClass(FlowCountMapper.class); job.setReducerClass(FlowCountReducer.class); job.setMapOutputKeyClass(Text.class); job.setMapOutputValueClass(FlowBean.class); job.setOutputKeyClass(Text.class); job.setOutputValueClass(FlowBean.class); FileInputFormat.setInputPaths(job, new Path(args[0])); FileOutputFormat.setOutputPath(job, new Path(args[1])); boolean res = job.waitForCompletion(true); System.exit(res ? 0 : 1); }
bin/hadoop fs -mkdir -p /flow/data bin/hadoop fs -put HTTP_20130313143750.dat /flow/data bin/hadoop jar ../lx/flow.jar
bin/hadoop jar ../lx/flowsort.jar cn/tf/flow/FlowCount /flow/data /flow/output
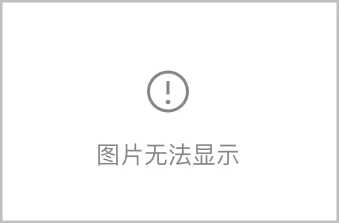
在这整过过程中,我们是有yarnchild的进程在执行的,如下图所示:当整个过程执行完毕之后yarnchild也会自动退出。
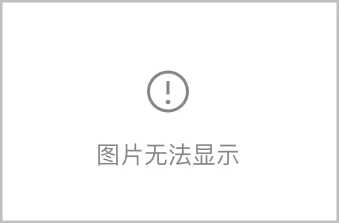
三、按总流量从大到小排序
如果你上面这个基本操作以及完成了的话,按总流量排序就非常简单了。我们新建一个FlowCountSort.java.
全部代码如下:
package cn.tf.flow; import java.io.IOException; import org.apache.commons.lang.StringUtils; import org.apache.hadoop.conf.Configuration; import org.apache.hadoop.fs.Path; import org.apache.hadoop.io.LongWritable; import org.apache.hadoop.io.Text; import org.apache.hadoop.mapreduce.Job; import org.apache.hadoop.mapreduce.Mapper; import org.apache.hadoop.mapreduce.Reducer; import org.apache.hadoop.mapreduce.lib.input.FileInputFormat; import org.apache.hadoop.mapreduce.lib.output.FileOutputFormat; public class FlowCountSort { public static class FlowCountSortMapper extends Mapper<LongWritable, Text, FlowBean, Text>{ @Override protected void map(LongWritable key, Text value, Context context) throws IOException, InterruptedException { String line=value.toString(); String[] fields=StringUtils.split(line,"\t"); String phone=fields[0]; long upSum=Long.parseLong(fields[1]); long dSum=Long.parseLong(fields[2]); FlowBean sumBean=new FlowBean(upSum,dSum); context.write(sumBean, new Text(phone)); } } public static class FlowCountSortReducer extends Reducer<FlowBean, Text, Text, FlowBean>{ //进来的“一组”数据就是一个手机的流量bean和手机号 @Override protected void reduce(FlowBean key, Iterable<Text> values, Context context) throws IOException, InterruptedException { context.write(values.iterator().next(), key); } } public static void main(String[] args) throws Exception { Configuration conf = new Configuration(); Job job = Job.getInstance(conf); job.setJarByClass(FlowCountSort.class); job.setMapperClass(FlowCountSortMapper.class); job.setReducerClass(FlowCountSortReducer.class); job.setMapOutputKeyClass(FlowBean.class); job.setMapOutputValueClass(Text.class); job.setOutputKeyClass(Text.class); job.setOutputValueClass(FlowBean.class); FileInputFormat.setInputPaths(job, new Path(args[0])); FileOutputFormat.setOutputPath(job, new Path(args[1])); boolean res = job.waitForCompletion(true); System.exit(res ? 0 : 1); } }
这个主要就是使用了FlowBean.java中的代码来实现的,主要是继承了WritableComparable<FlowBean>接口来实现,然后重写了compareTo()方法。
@Override public int compareTo(FlowBean o) { return this.sumFlow>o.getSumFlow() ? -1:1; }按照同样的方法对这个文件打成jar包,然后使用hadoop的相关语句进行执行就可以了。
bin/hadoop jar ../lx/flowsort.jar cn/tf/flow/FlowCountSort /flow/output /flow/sortoutput结果图:
四、按用户号码区域进行分类
流量汇总之后的结果需要按照省份输出到不同的结果文件中,需要解决两个问题:
1、如何让mr的最终结果产生多个文件: 原理:MR中的结果文件数量由reduce
task的数量绝对,是一一对应的 做法:在代码中指定reduce task的数量
2、如何让手机号进入正确的文件 原理:让不同手机号数据发给正确的reduce task,就进入了正确的结果文件
要自定义MR中的分区partition的机制(默认的机制是按照kv中k的hashcode%reducetask数)
做法:自定义一个类来干预MR的分区策略——Partitioner的自定义实现类
主要代码与前面的排序是非常类似的,只要在main方法中添加如下两行代码就可以了。
//指定自定义的partitioner job.setPartitionerClass(ProvincePartioner.class); job.setNumReduceTasks(5);
这里我们需要新建一个ProvincePartioner.java来处理号码分类的逻辑。
public class ProvincePartioner extends Partitioner<Text, FlowBean>{ private static HashMap<String, Integer> provinceMap = new HashMap<String, Integer>(); static { provinceMap.put("135", 0); provinceMap.put("136", 1); provinceMap.put("137", 2); provinceMap.put("138", 3); } @Override public int getPartition(Text key, FlowBean value, int numPartitions) { String prefix = key.toString().substring(0, 3); Integer partNum = provinceMap.get(prefix); if(partNum == null) partNum=4; return partNum; } }
执行方法和前面也是一样的。从执行的流程中我们可以看到这里启动了5个reduce task,因为我这里数据量比较小,所以只启动了一个map task。
到这里,整个用户流量分析系统就全部结束了。关于大数据的更多内容,欢迎关注。点击左上角头像下方“点击关注".感谢您的支持!
数据源下载地址:http://download.csdn.net/detail/sdksdk0/9545935
源码项目地址:https://github.com/sdksdk0/HDFS_MapReduce