登陆集群
1. 登陆集群
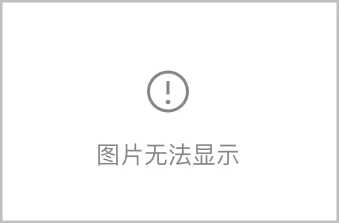
2.进入到EMR集群
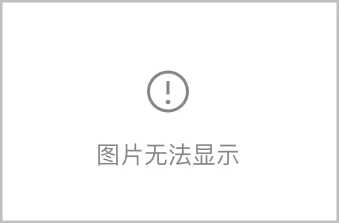
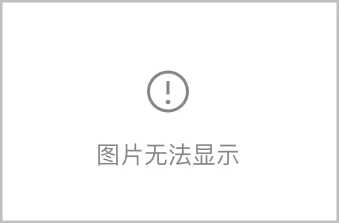
3.记住EMR集群的公网IP地址
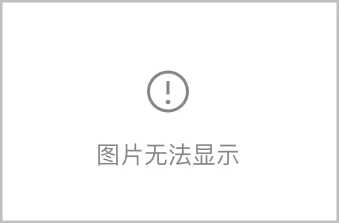
4.打开远程桌面终端
密码为 @Aliyun2021
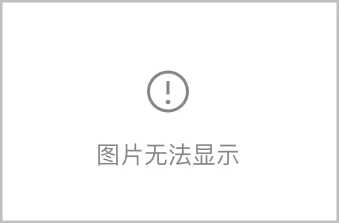
上传数据到HDFS
本步骤将指导如何将自建数据上传到HDFS。
1、执行如下命令,创建HDFS目录
[root@emr-header-1 ~]# hdfs dfs -mkdir -p /data/student
2、上传文件到hadoop文件系统
# a.执行如下命令,创建u.txt文件。
vim u.txt
# b.按 "i" 键进入编辑模式,通过粘贴快捷键(SHIFT+CTRL+V)将下方内容复制到文件中,按"Esc"返回命令模式,输入":wq"保存
# 说明:第一列表示userid,第二列表示movieid,第三列表示rating,第四列表示unixtime。
196 242 3 881250949
186 302 3 891717742
22 377 1 878887116
244 51 2 880606923
166 346 1 886397596
298 474 4 884182806
115 265 2 881171488
253 465 5 891628467
305 451 3 886324817
6 86 3 883603013
62 257 2 879372434
286 1014 5 879781125
200 222 5 876042340
210 40 3 891035994
224 29 3 888104457
303 785 3 879485318
122 387 5 879270459
194 274 2 879539794
291 1042 4 874834944
234 1184 2 892079237
119 392 4 886176814
167 486 4 892738452
299 144 4 877881320
291 118 2 874833878
308 1 4 887736532
95 546 2 879196566
38 95 5 892430094
102 768 2 883748450
63 277 4 875747401
160 234 5 876861185
50 246 3 877052329
301 98 4 882075827
225 193 4 879539727
290 88 4 880731963
97 194 3 884238860
157 274 4 886890835
181 1081 1 878962623
278 603 5 891295330
276 796 1 874791932
7 32 4 891350932
10 16 4 877888877
284 304 4 885329322
201 979 2 884114233
276 564 3 874791805
287 327 5 875333916
246 201 5 884921594
242 1137 5 879741196
249 241 5 879641194
99 4 5 886519097
178 332 3 882823437
251 100 4 886271884
81 432 2 876535131
260 322 4 890618898
25 181 5 885853415
59 196 5 888205088
72 679 2 880037164
87 384 4 879877127
290 143 5 880474293
42 423 5 881107687
292 515 4 881103977
115 20 3 881171009
20 288 1 879667584
201 219 4 884112673
13 526 3 882141053
246 919 4 884920949
138 26 5 879024232
167 232 1 892738341
60 427 5 883326620
57 304 5 883698581
223 274 4 891550094
189 512 4 893277702
243 15 3 879987440
92 1049 1 890251826
246 416 3 884923047
194 165 4 879546723
241 690 2 887249482
178 248 4 882823954
254 1444 3 886475558
293 5 3 888906576
127 229 5 884364867
225 237 5 879539643
299 229 3 878192429
225 480 5 879540748
276 54 3 874791025
291 144 5 874835091
222 366 4 878183381
267 518 5 878971773
42 403 3 881108684
11 111 4 891903862
95 625 4 888954412
8 338 4 879361873
162 25 4 877635573
87 1016 4 879876194
279 154 5 875296291
145 275 2 885557505
119 1153 5 874781198
62 498 4 879373848
62 382 3 879375537
28 209 4 881961214
135 23 4 879857765
32 294 3 883709863
90 382 5 891383835
286 208 4 877531942
293 685 3 888905170
216 144 4 880234639
166 328 5 886397722
# c. 上传文件u.txt到hadoop文件系统
[root@emr-header-1 ~]# hdfs dfs -put u.txt /data/student
3、查看文件
[root@emr-header-1 ~]# hdfs dfs -ls /data/student
Found 1 items
-rw-r----- 2 root hadoop 2391 2022-02-28 21:00 /data/student/u.txt
使用hive创建表
本步骤将指导如何使用hive创建数据表,并使用hadoop文件系统中的数据加载到hive数据表中。
1、执行如下命令,登录hive数据库
[root@emr-header-1 ~]# hive
which: no hbase in (/usr/lib/sqoop-current/bin:/usr/lib/spark-current/bin:/usr/lib/pig-current/bin:/usr/lib/hive-current/hcatalog/bin:/usr/lib/hive-current/bin:/usr/local/sbin:/usr/local/bin:/usr/sbin:/usr/bin:/usr/lib/flow-agent-current/bin:/usr/lib/hadoop-current/bin:/usr/lib/hadoop-current/sbin:/usr/lib/hadoop-current/bin:/usr/lib/hadoop-current/sbin:/root/bin)
SLF4J: Class path contains multiple SLF4J bindings.
SLF4J: Found binding in [jar:file:/opt/apps/ecm/service/hive/2.3.2-1.0.1/package/apache-hive-2.3.2-1.0.1-bin/lib/log4j-slf4j-impl-2.6.2.jar!/org/slf4j/impl/StaticLoggerBinder.class]
SLF4J: Found binding in [jar:file:/opt/apps/ecm/service/tez/0.8.4/package/tez-0.8.4/lib/slf4j-log4j12-1.7.10.jar!/org/slf4j/impl/StaticLoggerBinder.class]
SLF4J: Found binding in [jar:file:/opt/apps/ecm/service/hadoop/2.7.2-1.2.13/package/hadoop-2.7.2-1.2.13/share/hadoop/common/lib/slf4j-log4j12-1.7.10.jar!/org/slf4j/impl/StaticLoggerBinder.class]
SLF4J: See http://www.slf4j.org/codes.html#multiple_bindings for an explanation.
SLF4J: Actual binding is of type [org.apache.logging.slf4j.Log4jLoggerFactory]
Logging initialized using configuration in file:/etc/ecm/hive-conf-2.3.2-1.0.1/hive-log4j2.properties Async: true
Hive-on-MR is deprecated in Hive 2 and may not be available in the future versions. Consider using a different execution engine (i.e. spark, tez) or using Hive 1.X releases.
hive>
2、创建user表
CREATE TABLE emrusers (
userid INT,
movieid INT,
rating INT,
unixtime STRING )
ROW FORMAT DELIMITED
FIELDS TERMINATED BY '\t'
;
hive> CREATE TABLE emrusers (
> userid INT,
> movieid INT,
> rating INT,
> unixtime STRING )
> ROW FORMAT DELIMITED
> FIELDS TERMINATED BY '\t'
> ;
OK
Time taken: 1.016 seconds
hive>
3、执行如下命令,从hadoop文件系统加载数据到hive数据表。
hive> LOAD DATA INPATH '/data/student/u.txt' INTO TABLE emrusers;
Loading data to table default.emrusers
OK
Time taken: 0.47 seconds
hive>
对表进行操作
本步骤将指导如何使用hive对数据表进行查询等操作。
1、查看5行表数据。
hive> select * from emrusers limit 5;
2、查询数据表中有多少条数据。
hive> select count(*) from emrusers;
3、查询数据表中评级最高的三个电影。
hive> select movieid,sum(rating) as rat from emrusers group by movieid order by rat desc limit 3;